Flash and JavaScript are required for this feature.
Download the video from iTunes U or the Internet Archive.
Topics covered: Managing threats to evaluation and data analysis
- Attrition
- Externalities (spillovers)
- Partial compliance and selection bias
Instructor: Michael Kremer
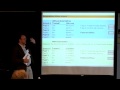
7: Managing Threats to Eval...
Related Resources
Lecture slides (PDF)
ANNOUNCER: The following content is provided under a Creative Commons license. Your support will help MIT OpenCourseWare continue to offer high quality educational resources for free. To make a donation or to view additional materials from hundreds of MIT courses, visit MIT OpenCourseWare at ocw.mit.edu.
MICHAEL KREMER: So I understand that in the session that you just had, you went through the deworming case. And I was just talking to some people in the break, and they were saying that everything's been very focused on methods, which is understandable. That's what the purpose of the course is. But it sounded like people were interested in hearing a little bit about the substantive results.
So I just thought before I launched into this lecture, I'd say a little bit about that. And maybe this is also a way to give you a little bit of background on where I'm coming from. So I taught secondary school in Kenya right after college and then went to grad school. And then I went back after graduating, from getting my Ph.D. and getting a real job and having some money. I eventually went back to visit some friends.
And one of them was working for an NGO, which was just starting work in western Kenya. And his job was to find seven schools to start a program in. And I said to him, not really thinking this was something that he would do, "Why don't you pick twice as many and choose the seven randomly, at least where you're going to start?" And much to my surprise, he was interested.
And then he went to his boss, and his boss actually did it. So that's, in part, how this wave of randomized evaluations with NGOs got going. This NGO worked a lot on education. And over the years, we tried a number of things to try to get more kids in school and stop kids from dropping out.
But eventually, they tried treating kids for worms. And part of this was based on reading the literature which suggested that this is an important health intervention. There was a question, would it have education effects. So it turned out, of all the various things that we looked at, we calculated what was the cost per additional year of schooling generated.
So we're comparing a bunch of things in that same environment in western Kenya. And deworming came out an order of magnitude better than anything else. So this was a really striking result. If you spent $3.50, you could generate an additional year of education for a child. It was just much cheaper than any of the other alternatives.
So we had that academic result. There were people at the World Bank who were very interested in this. There's a lot of heterogeneity in the Bank, but a lot of people there who are very interested in and understand evidence or are interested in or responsive to it. And the particular people who are working on health and education sector in the Bank in Kenya, that very much applies to. So they then took it to the Ministry of Education and brought us in to talk to the people in the Ministry of Education.
This process took quite a lot of time. I don't want to underestimate this. Took a lot of time. The first time, they said, these results are interesting, then, yes, we should pursue them. But there's a lot going on inside the Ministry of Education, lots of other priorities. There are teacher strikes. There's all sorts of things that have to take higher priority.
But both externally, outside in international fora and academic fora, and internally inside Kenya, we kept bringing this up. And eventually, the permanent secretary, who's very strong, the permanent secretary of the Ministry of Education said, let's do this. And he brought in various people. And they decided they were going to try to implement this, have a national scale up of this.
And there was both that internal persuasion of people within the Ministry of Education. And then, of course, there's the question of getting budget for it. Obviously, having the World Bank on side helped on that.
The other thing that we did was-- so Esther and I were both involved in an event the World Economic Forum put on in Davos. And working with that group, we were able to arrange for there to be an event in Davos on this issue of deworming. And we helped start an organization called Deworm the World which was designed to promote this.
And we invited the prime minister of Kenya to come speak. And he made this announcement. And I think that helped drive this forward a lot. Because once you got a public announcement by a politician, then it's really going to happen in a way.
So between the support internally within the ministry and this higher level political support, Kenya has, just as we speak, just in the past few months, dewormed almost 3 million children. So this is an example of how, if you can identify successful intervention, it can really help promote scale-up of the successful interventions. Ultimately, that's the purpose of what we're doing is trying to improve policy.
So I just wanted to give you that tie-in to reality before plunging back into econometrics. Any comments or questions on that?
AUDIENCE: I thought it was really interesting. Could you just give us a couple of the year points in that? You talked about things taking a long time. Can you give more concrete evidence?
MICHAEL KREMER: Our article appeared, and publishing the article takes a long time too. Our article appeared in 2004, I believe. It's now 2009, and this is happening now. The NGO responded much more quickly. Although eventually, they changed their strategy as well.
So the NGO scaled up. But to get the national government to scale up, I think that took a constellation of various people. It took some time for this to get in the media, to get in the academic world, to get out to the media, to get out to opinion leaders, both internationally. And then it took time for the right set of people to be available and the money to be available to do it. Yes?
AUDIENCE: Just kind of following up on that. This is a issue that is obviously quite concerning, the bridge between academia and the policy world. And the fact that this research is absolutely fabulous, but then, at the end of the day, it stays in a textbook. And what use is it to beneficiaries? This example is, again, fabulous. But what sort of actions or roles are there to extend findings into the policy world and into the development world? I'm sure that's a big topic. But just very briefly, is J-PAL or sister organizations doing that sort of extension?
MICHAEL KREMER: In this particular case, I would say that I spent a fair amount of time afterwards trying to disseminate this. And J-PAL has been very important in starting to deworm the world. I think this requires effort at a variety of levels, both in trying to get the prime minister on board, but also in trying to do tasks like, well, you need a spreadsheet of where are all the schools in the country, and which ones of them are in areas where we think that there's worms, and working out a bunch of logistics of, well, how many trainers do you need, and so on.
Now I think in different settings, they'll be-- in this one, people who are at J-PAL and IPA have been involved in even down to that spreadsheet level. That may not be the case all the time. I think it depends a lot on the particular government. I think, obviously, J-PAL is primarily a academic organization. And so it's not the right organization to manage the actual roll out. But where you draw the line, it's a difficult question. But I also-- Yes.
AUDIENCE: [INAUDIBLE].
MICHAEL KREMER: No, go ahead.
AUDIENCE: So it seems like that the deworming medication is really cheap, and it's a very easy treatment. Have you looked at other types of diseases and using the school system to try and manage it?
MICHAEL KREMER: Yeah. So there are other things which could be done in the school health area and perhaps with micronutrients, et cetera. I don't want to go too far there both because I want to get back to the econometrics and because I know more about worms than I do other things. But I think there are micronutrients and other things that could be delivered that way.
There's some work that's been done on presumptive treatment for malaria that is very intriguing and suggests that might work. There's also things you can do on education, HIV/AIDS education, and so on. Pascaline Dupas does some very nice work on that. And then Esther and Pascaline and Samuel Sinei and I have some joint work on that as well.
OK. Well, let me turn to the topic of this lecture, which is-- and I'm happy if there's time at the end or in the break, I'm happy to follow up on these issues-- which is managing threats to evaluation and to data analysis. So I think in the previous discussion, there's been things about how do you set up your sample size, how do you actually randomize.
Doing those things is obviously critical, but it might not be sufficient. Because there can still be problems with impact measurement and analysis. Some of those, you can try to minimize ahead of time. I'm going to focus mostly on what can be done ahead of time. And then Shawn's going to talk about what can be done in the analysis stage to try and deal with problems that did come up, and what inferences you can make, and what inferences you can't make.
I'm going to do a small, semi-randomized trial here, quasi-randomized trial, I guess should say. I'm going to consider a program which is giving people money as a social, anti-poverty program. I think, rather than do full randomization-- you can actually leave that down for awhile-- I'll come back to the evaluation of this program later. Now I'm going to do the randomization and the implementation. And we can do the--
AUDIENCE: Too late.
MICHAEL KREMER: OK. Too late? OK, that's fine. We could count off people, one, two, one, two, and then just give all the ones $500 and the twos nothing.
AUDIENCE: It really does feel bad when you're in the control group.
AUDIENCE: No sharing. [UNINTELLIGIBLE].
AUDIENCE: This is real money or this--
MICHAEL KREMER: Don't worry. Well, you shouldn't feel too bad if you're in the control group.
AUDIENCE: I meant cash or money?
MICHAEL KREMER: You've got the money now, yeah?
AUDIENCE: [UNINTELLIGIBLE] cash?
MICHAEL KREMER: Yeah, they'll be opportunities later on. OK, here are the problems I want to discuss. The first one is-- so hang on to this money, we'll deal with it later on-- the first one is attrition. The second is externalities. And the third one is partial compliance.
So the first one is attrition. Some people you're not able to collect follow up data on. You try, but you're not able to. The second one, externalities. What happens if your program, as in the case of deworming, winds up affecting the comparison group as well as the treatment group?
And the third one is partial compliance. You want to implement in certain places, but some places, they don't actually implement it. Maybe some of your comparison group accidentally gets treated. What do you do in that case?
All of these things are really about internal validity. So there's important questions of external validity and interpretation. And Shawn's going to talk to some about those. But I'm going to just focus on the internal validity of these.
So the first question with attrition is is it going to be a problem if some of the people disappear before you can collect the data. And this can be a real problem in Kenya for example. Kids often change their name. So it's just a part of the culture. You change your name at some point. That's going to make it difficult to find everybody afterwards.
So a first question-- oh gosh, I thought this was going to come up bit by bit. Well, OK. We got the whole slide.
So is it a problem if the type of person who disappears is correlated with the treatment? And does anybody want to answer that even though there's some answer there? This says the name of it, but it's not saying what the issue is. Does anybody want to comment on that? Yes.
AUDIENCE: So if the attrition is quantited with treatment, then you're going to end up with underestimated or overestimated effect depending on what the correlation is.
MICHAEL KREMER: OK. That's great. Can you say more about that?
AUDIENCE: So if the correlation is that the people who disappear are people who didn't get the treatment, who most needed the treatment, then what you're left with in the control group is stronger people, the people who maybe didn't need the treatment as much or maybe had other reasons that they were doing just fine. And so it's going to look like the treatment effect is less because you have a strong control treatment group compared to a randomized treatment group.
MICHAEL KREMER: Right. OK. So that's great. So let's go through an example where we can potentially see that sort of thing happening. So let's think about a problem where there's some kids who don't come to school because they're too weak, they're undernourished. So imagine that's the context. And imagine you start a school feeding program, and you want to do an evaluation of the impact of this on school attendance. So this, in fact, was something we wanted to do.
And imagine you're interested both in the impact on enrollment, but also on children's nutrition, which you measure by their weight. And imagine that the real effect of this program is that the weak, stunted children actually go to school more if they're near a treatment school. So if you go to all the schools and you measure everyone who's in school on a given day, in that case, are you going to see the treatment and control difference in weight overstated or understated?
AUDIENCE: Overstated.
MICHAEL KREMER: Overstated. So what's the story for why it would be overstated?
AUDIENCE: Because in the treatment schools, a lot of kids who really need the nutrition would start going in. Whereas, in the control group, they have no incentive to go. So they're not being included in it.
MICHAEL KREMER: That's interesting. That's interesting. OK, OK. In fact, the example is going to be the opposite. But I think it's true, you could tell a story where this could go either way. And you just told a story where it would go that way.
Let's show you a hypothetical numerical example. And if you can actually work through this, that would be useful. Imagine there's just three kids in each of these communities. So imagine that before treatment, the distribution looked identical. So there was one kid who weighed 30 kilos, another at 35, another at 40. And after treatment, let's say it's a successful program, and it gets everybody up by two pounds-- I guess we should do this in pounds given these numbers-- moves everybody up by two pounds. In the comparison group, everybody stays the same.
So when you calculate, the average here is going to be 35, and here it's going to be 35. So there's going to be no difference at baseline. If you look afterwards, if you didn't have any attrition and you managed to follow all these kids, you would correctly measure the impact of this program. You would say that it's added two pounds to people's weight.
Now here's one possible pattern of attrition. Suppose you go on a given day, but not all of the kids are there that day. So in particular, imagine that the weaker kids are less likely to be there. So suppose only children who are more than 30 kilograms come to school. Imagine the kids who are less than 30 kilograms are only there half the time or something.
And you happen to show up on a day when kids who are only over 30 kilograms come to school. Well, then the person who is still at 30 kilograms in the comparison group isn't going to be there at all. You'll measure the average here at 37 and a half. So you'll see no difference beforehand. Afterwards, can you compute what you're going to estimate the impact of the treatment to be? Yeah.
AUDIENCE: It's negative half a pound.
MICHAEL KREMER: Negative half a pound, right. So in this case, for this particular set of assumptions, you'll underestimate the impact. It's not necessarily the case that attrition differences between the groups always lead to underestimates. It can be the opposite. So we happened to pick a case here where it worked this way. But here's another example.
Let's put that other context behind us. Think about a different context. Think about the context of we're just trying to improve learning. And we've got a new math course. And it's a hard course.
For example, in the state of Massachusetts, there are now graduation requirements. Used to be that it was very easy to graduate from secondary school. They put in requirements to make this much tougher. You have to pass an exam.
And the proponents of this argue, well, it's a good thing because it forces the kids to study more, it forces the teachers to really prepare them. And they're probably right. The opponents argue that, well, the kids who figure they're not going to be able to pass just drop out. They may be right as well.
So if you're trying to evaluate the impact of this program-- and imagine that we randomized across states in the US, and some states implemented, and some didn't-- if you looked at the average score among those who got through, well, you might see it's better in the treatment group. But would that be the right conclusion about the impact of the program? It might not be. So let me keep going with this.
So we've got this harder course. Imagine those who can't handle it drop out. You give the same math test in the treatment and control schools. But you only have data on those who didn't drop out because you go to the school and you get everybody who's there in the school. So what's the direction the bias is going to be in that case?
AUDIENCE: It'll overstate the effect. You'll only see the strongest.
MICHAEL KREMER: Exactly, exactly. In the treatment group, you'll only see the strong students. In the comparison group, you'll have the mix. So that's an example of the case that you were talking about. In the deworming program with testing, what was the natural concern with attrition bias there?
AUDIENCE: The weakest, the ones with the most worms weren't going to be--
MICHAEL KREMER: Exactly, you get them to stay. The kid's pretty weak because they've had lots of worms. You cut off the worms, they come to school. So the treatment group would then be adding in these kids who are weaker in some way. So that would be the concern.
How do you deal with it? Well, one way is you can try to follow everybody up. And this is the first thing you should do is the brute force approach, which is to try and follow everybody up. And that means if it's a school program, maybe you don't just test the kids in the school, the ones who dropped out, you try and find them and test them anyway.
Now that's expensive. And it's very difficult to find people, and it may be difficult to get them to take the exam. But if you think that the program is going seriously affect dropout rates, then that can be a very important thing to do. To do that, you have to pick a sample of those who are going to be tested before the treatment, and you have to follow those people. So if you hadn't done a baseline, then this is going to be especially hard because you don't even know who dropped out. They might not have records of those kids.
There's sometimes questions. Should you do a baseline, or should you not? In theory, you could do a randomized evaluation without a baseline. Almost always, it's much better to have the baseline. And this is one of them, which is if the program might affect dropout, you want to measure the effect of the program by looking at the people who were initially in the program.
So then imagine that you do that, but the truth is it's just hard to find all these kids who dropped out. Some of them have moved, or they're not home, or whatever, or they don't want to come take the test. So imagine that you've done this, and the treatment group has 20% attrition, the comparison group has 20% attrition. Are you then OK? OK. I'm seeing the answer, no. Does anybody want to say what the potential problem might be? Yeah.
AUDIENCE: Well, if it's not random as to who drops out, then we're just still going to have to [UNINTELLIGIBLE] facts. If there's still a correlation between who's dropping out in the control group versus who's dropping out in the treatment group, that's still going to affect the outcomes.
MICHAEL KREMER: Yeah. That's exactly right. I'm trying to think of this myself. Can anybody come up with a hypothetical, but concrete example, where you could have the same attrition rate in the two groups, but your estimate would still be messed up or biased, to use the technical term? Yeah.
AUDIENCE: For example, if the treatment group is only the [UNINTELLIGIBLE] could drop off and then the control group is [? losing flow ?] would drop off, it's not going to [UNINTELLIGIBLE].
MICHAEL KREMER: Exactly, exactly. So if, in each case, you lose 20%, but in the treatment group, you're losing the top 20% and the comparison group, you're losing the bottom 20%, and you only measure those who remain, you're going to be biased.
So here's an example of something that could do that. Imagine that you put in a remedial education program. Imagine you lower the levels of the curriculum.
Well, then maybe the kids in the treatment group, maybe the kids who are at the top of the distribution say, I don't want to be in this school, I'm switching to another school, because they don't want the lower level curriculum. So you lose 20%. In the comparison school, the 20% at the top don't drop out, but the 20% at the bottom drop out because they didn't have this special attention. So in each case, you've got 20% attrition, but the estimate of the impact of the program is going to be very seriously biased.
So how can you deal with that? Well, what you should do is you should check whether you have a-- imagine you had pre-test scores for the kids. Well, then you could see what's the predictors of drop out in the treatment group and in the comparison group. And ideally, you'd find the predictors are the same. And then you're somewhat reassured. You're not completely, completely safe because maybe your initial test scores aren't really a good measure of their true eventual test score. But it helps a lot.
The other thing you can do is you can try to bound the extent of the bias. So we go through an exercise like this in the deworming paper. So suppose everyone who dropped out of the treatment got the lowest test score that you got. So what you can do is you can say, we're going to put those people for whom we don't have outcome data, we're going to create an artificial data set, where we put them back in the data, but we artificially assign them the lowest conceivable score.
And then suppose everybody who dropped out of the control group got the highest score that anybody could get. So if you artificially give everybody who dropped out of treatment the lowest possible score, and you artificially give everybody who dropped out of the control group the highest possible score, well, then you're bending over backwards to say, how bad could the program potentially have been. And if you do this exercise and you find that even when you do this, it looks like the program is good, then you can be pretty confident the program's good.
So this is what's called constructing the lower bound. And similarly, you can construct an upper bound on how well the program did. And if you have a high dropout rate, your lower bound and your upper bound are going to be very far apart from each other. You're not going to be able to say that much about what the impact of the program is. But if you have a low dropout rate, it might be that your bounds are very close together.
AUDIENCE: And cheaper.
MICHAEL KREMER: It's cheaper than fighting everybody, yeah. I think a lot depends on the particular context. And there's also various bounds you can do. So let me not go into the full detail on that. But you can have bounds that are very conservative. This would be an example of them, where this is very much a worst case scenario. You can imagine other scenarios that are not the very worst case scenario, but are pretty bad case scenarios, and say, even in that case, the program would have worked.
The next topic is going to be externalities. But before I go on to that, do people have questions on attrition or comments on it? Or questions about this in practice? OK, let me move on to externalities.
So first, I want to create some externalities. So everybody who got some money-- I heard a suggestion of sharing some money. So why don't we implement that? So why don't you turn to your neighbor, and why don't you share some of the money with your neighbor? I'll let you decide how generous you want to be. It is fake money after all.
AUDIENCE: Can we give to multiple neighbors?
MICHAEL KREMER: Do whatever you like, do whatever you like. And by the way, what you guys just did, there are a lot of theories of development-- I don't know whether this is practice or not-- which would say that that sort of thing might happen, a lot of theories about risk sharing within communities, and so on. Maybe that's all propaganda, I don't know. But anyway, some people would claim that that sort of thing can happen.
So now what I want to talk about though is what's the impact on our program evaluation. What I'd like to do is to do a program evaluation now of what was the impact of this program, where you're all a village. I gave half the people in the village $500. So how did we do that? Well, we pseudo-randomized the program, reasonably close, counting off one, two.
What's the impact of the program? Well, let's figure out how much money our treatment group people have and our comparison group people have. So if you can look in your wallet, figure out how much money you have there, add in the fake money, and come up with a total. And then we'll try and do some-- I'll do some data collection. So let me put this up here.
AUDIENCE: Our actual money?
MICHAEL KREMER: Yeah, add in your actual money and your fake money, and we'll see. So are you a treatment group person?
AUDIENCE: Yes.
MICHAEL KREMER: OK. So how much money do you have, including everything?
AUDIENCE: $784.
MICHAEL KREMER: $784, OK. I hope there's no thieves around here that I'm revealing things to.
AUDIENCE: $784?
AUDIENCE: Including this.
AUDIENCE: Because I'm a control group.
MICHAEL KREMER: You're a control group.
AUDIENCE: I have $300.
MICHAEL KREMER: $300.
AUDIENCE: [? Only money ?] pounds [? away ?].
AUDIENCE: But these guys gave it to you.
AUDIENCE: It was on your Charlie card or whatever.
MICHAEL KREMER: So how much do you have?
AUDIENCE: $407.
MICHAEL KREMER: $407. And you're a treatment, right?
AUDIENCE: I got $14 and $1 on my Charlie card.
MICHAEL KREMER: OK. So $15, we'll call it.
AUDIENCE: $550.
MICHAEL KREMER: $550. Maybe the second row should just come up and write on here.
AUDIENCE: $140.
MICHAEL KREMER: Sorry?
AUDIENCE: $140.
MICHAEL KREMER: $140.
AUDIENCE: $428.
MICHAEL KREMER: $428.
AUDIENCE: $318.
MICHAEL KREMER: $318.
AUDIENCE: $698.
MICHAEL KREMER: I'll put this here.
AUDIENCE: $263.
MICHAEL KREMER: And are you a one or a two?
AUDIENCE: I'm $500, I don't know what--
MICHAEL KREMER: So you're group one. And sorry, what was the number again?
AUDIENCE: $263.
MICHAEL KREMER: $263. You're a very generous guy at least with fake money, right?
AUDIENCE: $270.
MICHAEL KREMER: Oh, $270. Looks like the program was counterproductive in your case. We had a negative seven effect on income.
AUDIENCE: I have $227.
MICHAEL KREMER: $227.
AUDIENCE: $500.
MICHAEL KREMER: $500. You know what? We could go and do the full sample, but maybe we should-- well, we'll take two more.
AUDIENCE: $700.
MICHAEL KREMER: I'm sorry, which group are you?
AUDIENCE: Treatment.
MICHAEL KREMER: $700, OK.
AUDIENCE: I'm control, and I have $200.
MICHAEL KREMER: $200? Oh, so that got the-- We'll just take a partial sample rather keep going. Let's try and get the average in the treatment group and the average in the comparison group.
AUDIENCE: The average in the treatment group is 507 or 8.
MICHAEL KREMER: 508.
AUDIENCE: And the average in the control group is 249.
MICHAEL KREMER: 249. So now we do our evaluation. And we go through, and we say, OK, we gave out $500 to people. Now we've gone back to see how they're doing, compare them to the comparison group. And it looks like they're $259 richer.
So did the program work? Well, the program worked. But was it cost effective? Not really. Because we gave them $500. They're only $250 approximately richer. This really wasn't a big success.
AUDIENCE: Well--
MICHAEL KREMER: Go ahead.
AUDIENCE: That's only one way of looking at it, right?
MICHAEL KREMER: Exactly. That's one way of looking at it. If you came with that conclusion, you'd be missing a really important dimension of what the impact of the program is. Certainly, if you're a policy maker who's mostly concerned about what's the impact on the community, not what's the impact on the particular individual I gave it to, then you'd basically have a very misleading answer. So that's the danger. The topic of this lecture is what are threats. And this is a threat. You misunderstand the impact of the program because you haven't adequately accounted for the externality.
That's the problem. Let me now talk about what can you do about that problem. So let me look at this in the context of deworming. Then maybe we can come back to this example again. So in the case of deworming, a lot of the earlier work randomized deworming treatment within schools.
So the problem is that when you are dewormed, that may interfere with the transmission of the disease. If the treatment kills the worms in your body, that means the worms are no longer laying eggs, they're no longer being spread in the community as much. So what's the problem that that's going to create for the evaluation?
AUDIENCE: You're going to see benefits in the control group.
MICHAEL KREMER: Right. You could see benefits in the control group, just as this cash example. In this particular case, we argue that those benefits might not just have affected kids who go to that school, but might have also affected neighboring schools as well. But let's start out with the analytically simpler case. Suppose the benefits are local.
So suppose you only shared money with your neighbors, but you don't share money with people in another classroom in engineering or something like that. And how can you measure the total impact, the impact on the community as a whole of the program? What could you do in that case?
AUDIENCE: You could phase in at different rates to try and evaluate what would be the impact of just having a peer-controlled peer treatment and then try and figure out from the phase-in what the impact of the externality would be.
MICHAEL KREMER: So you could phase it in. In this case, if the externalities were local within a school or within a classroom, in the case of this money example, you could phase it in at the level of schools or of classrooms. And say, we're going to do 20% of the people in that classroom, 40% of the people in this classroom, 60% of the people in that classroom.
By the way, before I go further with this, so there's an advantage of this-- well, let me come back to this. I'm going to immediately assess the advantage of this, but there's also a disadvantage. So let's take this case where's there's externalities within a school.
So if we think about this particular case, so imagine that there's no externalities. Pupil one is treated, and the outcome is they don't have worms. Pupil two is not treated, but they still don't have worms. Some people just don't get the worms. Pupil three is treated. They don't have worms because the medicine worked. Pupil four is not treated, and they do have worms. Pupil five is treated, and they don't have worms. Pupil six isn't treated, and they do have worms.
So in this case, where there's no externalities going on, what's going to be the estimate of the treatment effect here?
AUDIENCE: 100% [INAUDIBLE].
MICHAEL KREMER: I'm sorry. You said 100%? Do you want to go through the reasoning you're thinking up on that?
AUDIENCE: [INAUDIBLE].
MICHAEL KREMER: So it's true that nobody who is treated has worms because the medicine works. So the total people in worms and the treatment group with worms is going to be 0. So in that sense, you've eliminated 100% of the group that does have worms. How many in the control group are going to have worms?
AUDIENCE: Three.
MICHAEL KREMER: Three. So it depends how you define-- this is a big distinction that people-- it's tedious, but it's important to make when you write things up is percentage effect versus percentage point effect. So percentage point is the absolute value. So let me first do the percentage point and then come back to the percent.
So we have 0 people having it in the treatment group. The total in the control with worms, was that three if I remember right? OK. So 50% of people have it in the control group, 0 have it in the comparison group. So it's a 50 percentage point difference. The difference between 50 percentage points and 0 percentage points. So one accurate way to write this up would be say we had a 50 percentage point difference. Another way would be to say, we eliminated 100% of the initial level. They're both accurate. It's just different ways of expressing it. When you write things up, the convention is to use percentage point for the absolute value. So the treatment effect would be 50 percentage points or 100%.
But now suppose that you actually do have externalities. So some children are not reinfected with worms. So these worms have a life cycle, so eventually the worms in you die. You have a high wormload because you're continually being reinfected. So think about this example, where some of the kids in the comparison group don't get reinfected. Let's just think about the percentage point effect for comparison. What are you going to estimate the impact being in this case?
AUDIENCE: 53?
MICHAEL KREMER: Right. Did I just-- let's see this thing. Let me just do the-- I'm sorry. We didn't-- I think this other thing, did we do the counting right in that one?
AUDIENCE: Well, you said there was 50% control with worms. And unless I'm misunderstanding it, it looks like it's 100%.
MICHAEL KREMER: Yeah, that's right. Somebody had said 50, and I didn't look. I just assumed that was the right number. Let me just look at the nos. Yes, that's right. I'm sorry. It's 100% who have worms. Sorry, that was very confusing.
So now I see why you said-- it's 100% either way whether it's percentage points or percent. You would have reached that same conclusion. Let me just go back here just to repeat, in case it wasn't clear to others like it wasn't clear to me. So if the total in the treatment with worms is 100% in this example, total in the control with worms is 0. I think I must've got confused in reading it in horizontal lines there.
So in this case, the total in the treatment group with worms is-- we still have 0 in the treatment group with worms. And in the comparison group, we've got 67%, 67%. So we're going to estimate the treatment effect in this case being 67%. Now notice that this--
AUDIENCE: 130? Why? Oh, because it's the difference.
MICHAEL KREMER: The difference, yeah. The difference between 100 and 67. Sorry, the hundred and-- see if we got this-- the hundred--
AUDIENCE: It's the total [UNINTELLIGIBLE] 0.
MICHAEL KREMER: This is 0, and this is 67. So we've estimated 67% effect. So the thing to take away from this is that if there were no externalities, we would have estimated this correctly at 100%, the effect of the program.
Now we say, suppose there are externalities to this. So now that makes the program actually better because more people are being cured of worms through this program. But we're going to estimate the effect of the program is actually lower. Instead of estimating the 100% benefit, we'll estimate only a 67% benefit.
So how do you deal with that? Well, if you design the unit of the randomization, so it encompasses all those spillovers, that's one way to address this problem. So if you expected all the externalities are within school, you can just randomize at the level of the school.
So here's another approach. And this is the actual data from the program. The percentage of children with a moderate or heavy infection in the treatment schools was 27%. It was 52% in the comparison schools. So the program reduced moderate to heavy infections by 25%.
This medicine probably affected more kids initially. But if you go back and measure a year later, some of them have been reinfected. You also got a reduction in the number of kids who were sick and who are anemic. This is comparing one school to another school. So we will have accounted for the total impact of the program within schools if there's within school spillovers.
Suppose you wanted to actually measure the spillovers. Suppose you were interested in the spillovers themselves and not just the total impact. And you might well be. Imagine you are interested in the question of do we really need to incentivize people to take this, or could we charge them for the medicine. Well, if you thought that everybody benefited from the medicine pretty much equally, whether you took it or not, because most of the impact was on the transmission of the disease, then you might need to subsidize it more. You might want to subsidize it more than if you thought the individual got all the benefit.
So if you actually want to measure the spillovers, here's one of the things we did in the paper on deworming. So at the time-- this is no longer the case, I want to emphasize-- but at the time, there was concern that the official guidelines were not to treat girls over 12 unless you knew they had worms, they shouldn't be treated presumptively in case the medicine caused birth defects and in case the girls were pregnant. Turns out, that they've now given this widely enough that WHO guidance is there's no evidence it causes birth defects, and you can give it to everybody. But at the time, they weren't giving it to girls above 12.
So imagine you compared girls above 12 in the treatments schools to girls above 12 in the comparison schools. There are some other things we can do. So there are some other sources of who wasn't treated. This comparison I'm going to show you is a little bit more than that. But you can compare the treated students in treatment schools to the comparable students in the comparison schools.
So kids who looked comparable on a variety of observable dimensions or who wound up taking this when they became eligible to take it. We saw a very big gap in prevalence among those two groups. So this is much more of a straight treatment comparison look. Here, we're looking at the untreated students in the treatment schools and trying to find comparable students in the comparison schools.
I should emphasize this isn't quite as pure as a standard randomized design. This program was phased in over time. These are the people that when their school was phased in, they wound up not getting treated. So maybe there were differences between years, but that's a caveat or a footnote. So none of these guys were treated, but these people were in a school where their classmates were treated. So they have much lower levels of infection than these people who were also not treated, but whose classmates were not treated.
Now what if you expect externalities across-- so actually, before I go on to this further challenge of what if there are externalities across schools, just sticking with this question of externalities within schools, talked about one way of dealing with that was to do the randomization at the level of the school. So what's the disadvantage of doing the randomization at the level of the school?
AUDIENCE: Assuming that everybody in the same school is at the same level.
MICHAEL KREMER: So you could still have some differences within the school. But there is a sense in which you're going to have less information if you're randomizing at the level of the school. Yes.
AUDIENCE: You'd need more schools.
MICHAEL KREMER: Yeah, right. The crudest way of putting this is if there's 400 kids in a school and you have 100 schools and you're randomizing at the level of the individual, then you've got 40,000 observations. And if you've got 200 schools you're randomizing and you're randomizing at the level of the school, you've got 100 treatment schools and 100 comparison schools. Much smaller sample size, much less power.
That particular calculation I just did is overstating the difference. You've learned about clustering standard errors before. But since there's a lot of variation-- to come back to the way you were putting it-- since there's a lot of random variation between schools-- some schools have good headmasters, some schools have bad headmasters, et cetera-- there's a lot of background noise. And it's going to make it harder to estimate precisely the impact of the program.
So you really have to think about your particular context. When you're thinking about what level to randomize at, think about, in your context, do you think spillovers are a real issue. If you think spillovers are a real issue, then you better randomize at a higher level. But if you think, in this particular context, I don't need to worry about it, if this were a cancer drug rather than a worm drug, then you wouldn't need to worry about it, and you're much better off randomizing at the individual level. There might also be-- yes.
AUDIENCE: So this might be a little too [INAUDIBLE], but if you're worried about attrition, would randomizing at a higher level make that less of an issue as well because now you're looking at a higher [INAUDIBLE]? So if you lose individuals within that, it's still an issue.
MICHAEL KREMER: It would still be an issue. Yeah, it's still an issue.
So let's say that we've decided we're going to randomize. Take this worm example. And we think that most of the externalities are within schools, so we're going to randomize within schools. We know that there might be some externalities across schools because this is an environment where everybody lives on their own farm basically. So you might have two kids living next to each other, one of whom goes to one school and another goes to another school. That's not that uncommon.
So you could have some externalities across schools. But randomizing at the level of a district would really not logistically be very possible. You'd have no sample size left. So you know there might be some externalities across schools as well as those within them.
But you've already made the decision to randomize at the level of schools. So what do you do? Well, what you can try and do is use random variation in the density of treatment nearby. So if you pick the schools randomly that were going to be treatment schools and the ones that are going to be comparison schools, there will be some comparison schools that happen to be completely surrounded by treatment schools. There will be other comparison schools that don't have any treatment schools nearby. So you can use that to try to pick up how big the externality is. So that's what we tried to do in this paper.
So here's a map. So the ones are group one schools. They've been treated. The twos are group two schools, treated in the second way. Threes are group three schools, now treated to the third way. Here's a school that's in the middle of the lake which I think is actually on an island.
These schools in Uganda are not really in Uganda. GPS used to be intentionally degraded because people were wanting to use it for military-- it was developed by the military, I guess-- they didn't want foreign militaries to have it. There are measured with some error.
So we've got these schools. So we can see there are some schools that are near treatment schools. Other schools aren't near treatment schools. By the way, the treatment schools in this example I just did here, the ones shared with the twos. But you could imagine the ones might share with other ones. So there could be externalities on other treatment schools.
Here's a group three school that's all by itself and doesn't have any neighbors who were treated. Here is a group three school that has three group one schools that are treated. So would you want to compare those to estimate what the effect of the deworming program is?
AUDIENCE: Wouldn't you use it to estimate the impact of the spillovers?
MICHAEL KREMER: Yeah, suppose you were interested in estimating the impact of the spillovers, the medical spillovers of treatment. Could you compare those two schools? What might make that comparison invalid if you're trying to estimate the impact of spillovers?
AUDIENCE: [INAUDIBLE].
MICHAEL KREMER: I'm sorry. One means a treatment school. Three means a comparison school.
AUDIENCE: [INAUDIBLE].
MICHAEL KREMER: So one's more rural. Exactly. This one is obviously in a less densely settled population. This one, turns out these are all rural, but this is obviously much more densely settled. That's why they've got all these schools around there. So now in this particular setting, why might that be a problem? Yeah.
AUDIENCE: Because that area might be internally different from the [INAUDIBLE].
MICHAEL KREMER: Yeah. So this is a disease. I probably should have said more about this in the beginning. So these worms affect one out of every three or four people in the world. And they're spread through fecal-oral routes. They're spread through fecal matter.
What are the odds that you're going to get contaminated with fecal matter? It depends how many other people are depositing fecal matter in the environment. Clearly, over here, there's a lot of people nearby you who might be depositing fecal matter in the environment. Over here, there aren't so many.
So you might think that that's how densely settled the population is. We don't think of Alaska or the middle of the desert somewhere being very diseased environments. But you think of a highly concentrated place having a lot more disease. There's reasons to think that sparsely settled places will have different prevalence of the disease than heavily settled places.
So what we did because of that, we didn't want to just look at the number of treatment school nearby. We just talked about why that would be a problem. But we want to do that controlling for the total number of schools nearby. So we control for the total density in the area, total number of schools within a certain distance or pupils within a certain distance, and see what's the effect of those schools being treatment schools as opposed to being comparison schools. Oops, did I skip a-- OK.
So controlling for density, what we find is that the infection rates are 26 percentage points lower per 1,000 pupils in treatment schools within 3 kilometers. And then if you go further out, there are 14 percentage points per treatment schools that are 3 to 6 kilometers away. So this is controlling for the overall density in the area. So hopefully, we're abstracting from that particular problem.
So now suppose we want to estimate the overall effects. So let me come back to this problem. Clearly, we've incorrectly estimated. We estimated that only $250 of benefit went through. But we think that the true effect should include the effect on the comparison.
In this previous case, we were able to estimate the increase in school participation in the treatment group and then also in the comparison group through this technique that I just outlined. So we know in the comparison schools, there's a 1.5 percentage point increase in school participation. There are three pupils in control schools for every treated child. And in the treatment schools, there was a 7 percentage point increase in school participation for all children, but you only needed to treat 2/3 of the children. So you can then calculate what the overall effect is of treating one child.
So if you treat one child, you pick up three children in comparison schools, each of whom gets a benefit of 0.015 additional years of education. Then you pick up this is the effect on children in the same school, and you get an overall effect of 0.15 years of education. So treating a child costs about $0.50-- in fact, it's probably cheaper than that when done at scale-- but the impact that you're going to get is if each child, you get an extra 0.15 years of education, if you treat seven children, you'll get about an extra year of education. 7 times $0.50 is $3.50. You spend $3.50 on deworming, and you get an extra year of education.
Let me pause again here, and I'll go on and discuss some issues on partial compliance and sample selection bias. I'll get partway through that topic, and then Shawn's going to take up where I leave off. But are there any questions on externalities before I go on? OK.
So you might think if you randomize where the treatment is, you're going to get rid of sample selection bias. That's not necessarily the case. So let me show an example. So where you randomize where you want the program to be, that's not necessarily the sole determinant of which places actually get treated. So let me talk about why.
So one example would be people who are assigned to the comparison group might try to move into the treatment group. I don't think this happened, but parents could try and move their children from the comparison school to the treatment school. It's at least hypothetically possible. What are other possible reasons why you might not get this match between the initial assignment and where people wound up, where the people wound up treated? Yeah.
AUDIENCE: So you might get somebody treated, and they don't want to take the medication.
MICHAEL KREMER: Sure, exactly. In the case of deworming, there were some people who either didn't want to take the medication or who maybe they wanted to, but they weren't able to get the permission slip to do it. So that's one great example. What are other possible examples?
If you think about your concrete experience, imagine that-- I'll tell you a story from our experience. When this NGO was trying to get started and they were trying to pick the seven schools where they were going to work, they picked the schools, and they had to go to the government for permission to start working. And permission was slow. It kept being slow and slow. And they didn't realize what was going on.
And it turned out, eventually, that there was a politician who was upset. The NGO didn't understand why the politician was upset. Because one of the schools was in his constituency, where they were going to start working. Well, it turned out it was in the part of his constituency that voted for his opponent.
So in that sort of a situation, what the-- I don't remember exactly the timing of this, but what the eventual resolution of this was they started working in the other part of his constituency, where his supporters lived as well. So they're all sorts of cases where you're going to want to randomize, but you may not be able to have that happen perfectly.
In this case, it wasn't a, quote, "legitimate" reason. But there are other cases where there'd be very legitimate reasons why. Maybe the need is very intense in some area, and so the NGO or the organization feels it's very important to work in that area. So there may be lots of reasons why some people in the comparison group wind up getting treated.
So there are cases like we just heard about where individuals allocated to treatment might not get treatment. And there are cases where people who are in the comparison group do get treated. So in the case of deworming, 78% of those assigned to receive treatment got some treatment. And the main reason they weren't treated is they just happened to be absent from school the day that the treatment was given. Some students in the comparison group were treated because they went out and got the treatment on their own through clinics.
So what do you do? Suppose this has already happened. So imagine you have data on everybody, so attrition isn't the problem, but just the actual assignment to treatment. The assignment to treatment and actual treatment don't correspond. So first, what's the problem if you just do a straight comparison, and what might you do about it?
AUDIENCE: In this [UNINTELLIGIBLE], say they [UNINTELLIGIBLE] the students who were absent just by [UNINTELLIGIBLE] to their homes and [UNINTELLIGIBLE]?
MICHAEL KREMER: No, so the program didn't do that. So we talked about in the case of the evaluation, when you're trying to measure the test scores or the impact on attendance, you could-- well, impact on attendance, obviously, you find out whether they're there or not by visiting the school. Unless you wanted to do test scores, you could track them home. But the way the program was implemented, those kids who weren't at school that day when they gave out the deworming pills, they just didn't get treated. Maybe the program shouldn't have been run that way, but that's how it was run. And there are reasons why maybe it should be run that way.
AUDIENCE: In the end, you wouldn't be considering the effect of actually treating people? You'd be comparing the effect of intending to treat people.
MICHAEL KREMER: This is exactly where we're going to go, and it's where I'm going to wind up and where Shawn's going to be taking over. Imagine you are interested in the impact of this program on test scores. So one thing you might think would be the right thing to do would be to just look at the people who actually were treated and compare them to people who actually weren't treated. That's going to be problematic for reasons that we'll explain later.
But let me follow up on your suggestion which is if you're a policy maker, there are questions beyond this you'd be interested in. But you raised the idea of saying, well, what's the impact of the intent to treat somebody. And that is going to be the right answer to some questions. So let me start with that question, the relatively easier question. I'll let Shawn handle the harder questions.
Suppose you're a policy maker, and you're saying, look, what's the impact of putting in this school-based deworming program? Well, if you're interested in what's the impact of a school-based deworming program, well, you know in reality, it's a true thing that you want to get at that some people are not going to get it. If this is a school-based program and you hand out the pills at the school, tracking kids to their houses who are absent that day, that's expensive. That's hard to implement. It uses too much teachers' time. You're probably not going to find that many of the kids anyway. So you wouldn't actually implement it that way.
If you're a scientist, you do care. But if you're the policy maker, you might say, no, the true effect of this program is that I'm only going to be able to get 78% of the pupils because 22% of the pupils aren't there. And if some kids don't want worms-- don't want the medicine-- sorry, if they don't want-- or maybe they do want the worms, or they don't want the worms, but they don't want the medicine either. Anyway, if some kids aren't going to take it, then those kids aren't going to take it.
So you might think, well, I want to measure the impact of this program in realistic conditions. And realistic conditions are that not everybody's going to be able to get it. So let's suppose that you're a policy maker. Then what you could do is you could look at what's called the intention to treat estimate, which is what's the effect of the school having the program or being assigned to the program.
This comes up in medical trials a lot with, say, chemotherapy. So some people who start chemotherapy don't finish it because it's just too painful for them. Or they're not able to handle it medically. Again, do want to measure the impact of chemotherapy on those people who managed to get all the way through? Well, not necessarily. Maybe what you're interested in is what's the effect of being in this group that tries it. Yes.
AUDIENCE: Do you think it actually happened in 1997?
MICHAEL KREMER: So yeah, I guess that actually helps on the dates.
AUDIENCE: So it actually is 10 years on the--
MICHAEL KREMER: That's right. So it's 10 years. It's 10 years before this was rolled out nationally. So yes, some things happened before that, but this is a long delay. Yeah, so that first delay of publication took quite a while. And then there was a second delay after it. Unfortunately, there's often a long delay in these things. Let me see where we are.
So what you can do is you use the original assignment, and then you're winding up with what's called an intention to treat estimate. So what intention to treat measures is what happened to the average child who was in a treated school in the population. So it's not saying, what happens to the kids who actually got the medicine. It's saying, what happened to the average child who is in a treated school. So that's the correct interpretation of that.
Now is that the right number to look for? I talked about some purposes where that might be the right number to look for. What would be some reasons why you might be interested in other questions other than the answer to that question of what happened to the average child in a treated school?
AUDIENCE: You were thinking of having a mandatory deworming program in Kenya. And then you want to know what would be the impact if everybody was forced to treat.
MICHAEL KREMER: Exactly. So in this particular case, this was a program where it was designed in such a way that not everybody had to be treated. It wasn't that you can't come to school unless you show your certificate showing you've been treated. But you might well be interested in, well, what if we went a step further. And we said, we're going to keep a supply of medicine at the school, and if you are gone that particular day, then you get it the next day. And we don't let you come back to school unless you take the medicine. Well, you wouldn't be measuring the impact of that program.
Intention to treat is very good if you're interested in the narrow question of what's the impact of this exact program. But if you're trying to go beyond what's the impact of this exact program, you're trying to start to think about generalization, then maybe you want to understand some of the underlying parameters. And in this case, the underlying parameter is what's the effect on school attendance of a kid who had worms or a particular level of worms no longer having that. And then it's using that underlying parameter that you might be able to generalize what would be the effect of everybody getting treated, what would be the effect of only some people getting treated.
So to do that, Shawn's going to talk a little bit about how you would do that. Let's do this example, where we're trying to get the-- I'm wondering whether to skip this example or not. I'll do it. I'll go through it.
In this example, if you look at the people who were-- here's the intent, whether there was an intention to treat them. So all school one, you tried to treat everybody, but only some of them got treated. In school two, the intent was not to treat that. They were assigned to the comparison group. But a few people got treated anyway.
And this is the change in weight for each individual. So then if we average the change in weight, the average change in school one-- I don't know if people want to figure that out for a second--
AUDIENCE: [INAUDIBLE]?
MICHAEL KREMER: Sorry?
AUDIENCE: [INAUDIBLE]?
MICHAEL KREMER: So it's 1.3, right? And the average change in school two is 0.9. So the intention to treat effect would be comparing the 1.3 to the 0.9. Now when is that useful? Well, that's what I'm saying, for an actual program. But you're not measuring this medical effect that you'd want for generalization.
Here is an example where it's a malaria prevention program, but there's political pressures to treat. And so you add. Again, you can measure this impact, this intention to treat measure. Let me-- I'm wondering whether I should-- let me go back here and say--
Initially, the blue circles were the ones that were supposed to be treated. I want to talk about why you can't do what the apparently obvious thing of just comparing the guys who were treated to the ones who weren't. You've got this malaria prevention program. 40 villages are sampled. 20 were assigned to get the treatment the first year. 20 were assigned to be the comparison.
But some of the comparison villages object to this, and they say, we want to be treated too. And the program manager says, look, we just have to go ahead and treat this. So if the program only gets implemented in 15 villages, as well as in 2 villages that were supposed to be comparison, so what do you do to measure the impact of the program?
So by the way, in the previous case I mentioned with the politician in Kenya, the extra school was neither treatment or comparison, so really, in that case, there was no problem because it was just out of the sample frame altogether, the extra school that got treated. In this case, some of the comparison schools wind up getting treated.
So how do you measure it? Well, here's the problem with what would happen if you just did the naive thing and said, we're going to compare all the guys who actually got treated to the comparison schools.
So we've got the blue schools are the ones that were supposed to be treated that are in the sample. The white schools are other villages. So T is the original treatment group. The T's are supposed to be treated. The blues without T's in them are supposed to be the comparison. Now the actual treatment are the green circles.
So you can't compare the green circle villages with the blue dots. The green circles are the ones that were actually treated. And the blue dots are the comparison. Why can't you make that comparison?
AUDIENCE: They're not randomly assigned from the very beginning.
MICHAEL KREMER: They're not randomly assigned from the very beginning. And can you be more specific about what your hypothesis might be on the difference?
AUDIENCE: [UNINTELLIGIBLE] that fought to get the treatment would prefer some help from the schools or villages that were initially selected randomly.
MICHAEL KREMER: Exactly, exactly. The guys who fought to get the treatment might differ from the ones that are initially selected randomly. They might have particularly capable leaders, for example, or influential leaders. And those influential leaders-- this politician who managed to get the NGO program assigned to his area, he might have fought to get lots of other programs assigned there. So we don't know whether we're measuring the impact of this program, or we're measuring the fact that they're just able to use their political influence to get everything assigned there. And similarly, if you leave out the--
So this is basically just making the point that you said. The other thing that you could think about doing is comparing the villages that were assigned to be a treatment group and actually got treated with the ones that were supposed to be a comparison group. So what's the problem with that?
AUDIENCE: Attrition. It's kind of like attrition.
MICHAEL KREMER: Exactly, it's the same principle, which is you'd be leaving out a group that is the ones who were assigned to be treated, but didn't wind up getting treated. Well, the ones who were assigned to be treated and, nonetheless, didn't get treated, those might be the ones-- so for example, imagine there's violence in some of these areas. And your field workers can't go there, so they never wound up getting treated.
Well, the violence itself might have had an impact on development outcomes. So you may be measuring the impact of the violence or of particularly bad leaders who, despite being in the treatment group, still can't get their village treated. So that's not going to be a valid comparison either.
So one thing you can do is the intention to treat estimator. You can do that again in this case. So compare the initial 20 treatment villages with the initial 20 comparison villages. And then you've got the ITT estimator.
Now before I argued that the ITT estimator, in the case of the deworming program, that arguably might be a very good measure of some things. You might not be able to do some other things with it, but it was still a useful measure. But in this case, suppose we want to actually understand what the impact of the malaria treatment program. And we know that what's the impact of it if you're able to implement it. Well, the intention to treat estimator isn't really telling you that. It's telling you what's the effect of being assigned to the treatment.
But it's not saying what's the effect of the program in the cases where you're able to implement it. So that's a problem. And that's where I'm going to leave you with that problem. And then Shawn's going to tell you, at least, a solution to it.