Flash and JavaScript are required for this feature.
Download the video from iTunes U or the Internet Archive.
Topics covered: Why randomize?
Instructor: Dan Levy
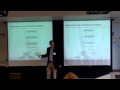
2: Why Randomize?
Related Resources
Lecture slides (PDF)
SPEAKER: The following content is provided under a Creative Commons license. Your support will help MIT OpenCourseWare continue to offer high-quality educational resources for free. To make a donation or view additional materials from hundreds of MIT courses, visit MIT OpenCourseWare at ocw.mit.edu.
PROFESSOR: OK. So before we begin, I would like to just ask a very simple question. Do you think randomized evaluation are the best way to conduct an impact evaluation? Please raise your hand if you think so. Just be honest. All right, the TAs, you guys don't count.
All right. OK. So I have a job to do now. Whereas I thought that maybe not.
One of the things I would like to do is to-- this is one thing I've discovered about teaching. We have about an hour and 25 minutes. And if I speak for an hour and 25 minutes, I know two things will happen. One, you will get very bored, and two, you will not learn anything. So I want you to make sure that you interrupt with questions that you have. If they can be on the topic, that would be very good. If they're are off-topic, I may delay the question or I may postpone the question, at least until I get there.
The other thing I would like to say about the way this would work, is I have no power over you. Whereas my students, I have a grade to give, with you I have no power. But I will still ask you to do certain things during the presentation. So I hope you'll collaborate.
So my session is called Why Randomize? And the idea of Why Randomize? comes, for those of you who are convinced, I hope you can use this session to help convince others why this method is a very good method to do an impact evaluation. And for those of you who are not convinced, I would like to actually welcome you to raise any objections you have. And I'm not here to tell you randomization is a panacea or it's a solution to all the problems of mankind. But I think in terms of impact evaluations, it's a very powerful method.
So the outline of the talk, I'll give you a little bit of background. We'll define, what is a randomized evaluation? It's going to be important to make sure we have a common language. Then advantages and disadvantages of experiments. Then we're going to do the get out the vote, and then finally conclude in hopefully an hour and 20 minutes.
So how to measure impact? This is something that Rachel referred to. The idea for measuring impact is, we want to compare what happened to the beneficiaries of a program versus what would have happened in the absence of the program. This is really key. What would have happened in the absence of a program is what's called a counterfactual, and it's key for you to evaluate any method to estimate program impact. Not just randomized evaluation.
So when you are trying to assess how someone is going to do an impact evaluation, always ask yourself the question, what is the counterfactual here? How are they planning to think about this counterfactual? How do these people look like in the absence of the program?
In the case of Kenya in the textbooks that Rachel was referring to this morning, we thought about the counterfactual in terms of how these children fared after this textbook program was implemented versus how they would have fared at the same moment in time had the program not been implemented. This is crucial, because even before and after methodologies or any other of those methodologies, you are assuming implicitly counterfactual.
And the question is, what counterfactual are you assuming, and then is that assumption realistic? And in some cases, it may be. In other cases, it may not.
So the problem is, the counterfactual is not observable. So the key goal of this impact evaluation methodology is to mimic it. You can't observe how this children in Kenya would have fared if the textbook program had not been implemented. The truth is, the textbook program was implemented, these textbooks were sent, and so you can't observe what that alternative reality would have been. And so constructing the counterfactual is usually done by selecting a group of people-- in this case, children, in the case of the Kenya example-- that have not been exposed to the program, or were not affected by the program.
And so in a randomized evaluation, the key goal here of the randomized evaluation is that you do it from the beginning. And this is a question that I think Logan had in the first session with Rachel. You can't do a randomized evaluation three years after the program was implemented. And the reason you can't do it is that you need to create, through this randomized experiment, the treatment in the control group. You need to decide early on who's going to get the treatment or who's going to be offered the treatment and who is not going to be offered the treatment.
There are some opportunities, as Rachel referred to, and your get out the vote case is a good example, where someone already did this. And so you may be lucky, and you may step into the room and say, oh, look. Someone did it. But this thing is, someone should have taken care so that the assignment to this treatment and control group was done in a random manner.
And in effect, and we'll see what exactly is random, but what I can tell you for now is if someone doesn't say, we did it randomized, we did a deliberate process so that it was random, it's probably not random. Random is not what people say in the real world. Oh! This is just a random event. Random has a very specific definition which we're going to see in a second. So it's not enough to just say, oh, look. We didn't do anything systematic. Just people enrolled, and that's what happened. If they didn't do something deliberate to do it random, then it probably wasn't random. You can try to check this, but not always possible.
The non-randomized, basically, I use that some excluded group, the group of people you're going to use as this comparison group, it's mimicking this counterfactual. And the non-randomized methods rely on the strength of the assumption that you're making. So the methods will be strong if the assumption that the comparison group mimics the counterfactual is a good assumption. There's not any sense in which you say, well, this method is better than other this other in some absolute manner. It is better or it's not better if the assumptions needed to mimic the counterfactual hold. If they hold, then that's great, you have a good method.
The key distinction between this-- yes?
AUDIENCE: Could you give us an example of when the assumptions were just obviously untrue?
PROFESSOR: Sure. So suppose that you had this textbook program and it was happening in Kenya, where many-- and this is program happened-- where many other things were happening in this education system. So textbooks were being distributed, different teachers were being hired. A lot of activities were happening.
And so you just compare what test scores of children were before the program and then what textbooks of children were after the program, you would suspect that-- well, first of all, if you did that, the counterfactual you would be assuming is that in the absence of the program, test scores would have remained flat. And that may be a reasonable counterfactual in some contexts. Not many, to be honest. But not in others.
So in one context in which other things happening in the education system in Kenya, it's very hard to argue that nothing would have changed in test scores. Because test scores would have increased, because there are lots of things that happen.
Now suppose you implemented this same program in a very remote village, very secluded area where nothing else would have happened. You sort of have a pretty good sense that no other intervention was happening for one group or the other at the same time. The assumption maybe more plausible. I think in this case, the textbook example, it's still questionable, because there are other educational input said may be happening.
But the key is that the context and the method are the ones that together can tell you how good the assumption is. The method by itself cannot tell you. The method by itself may be reasonable under certain conditions but not under others.
AUDIENCE: But there aren't any sort of big famous studies that weren't randomized, that everybody thinks they're pretty good?
PROFESSOR: Yes. So I don't want to get a lot into this, but there's a whole debate now in economics literature as to whether randomized experiments are the only way to estimate causal effects. This is a big, big debate, and there are very respectable people on both sides of the debate. What I can tell you is that debate has not been solved, but I think more and more people are sort of recognizing, at least, that the randomized experiment should be a first best. I think even the opponents of the method do say that.
But the other thing I would say is there have been many studies trying to compare the results of an experiment with some of the other non-experimental methods. You have one in your get out the vote. That was not a study in which the non-experimental methods fared very well, but there are other studies in which they fared well.
The key thing is we haven't been able to figure out under what conditions the non-randomized evaluations fared well. If we knew, then it would be nice. But I think so far, the answer-- we don't know. We know the theoretical answer, which is, if the assumptions hold, we're golden.
The problem, key problem, is that this is relying on the assumptions, and you cannot test these assumptions. If you could test this assumption, if you could test under what assumption this mimics the counterfactuals, we'll be all done. We'll be able to say, from the very beginning, we can use this method. You cannot, no matter how sophisticated and how good the non-experimental method is.
Yes? You seem skeptical.
AUDIENCE: No, no, no.
PROFESSOR: You're--? OK.
So this is very confusing. It's like twice they're showing-- you should do a randomized evaluation to see if this helps. Two boards.
All right. So the randomized evaluations here, you have a bunch of other names in which they are known-- random assignment studies, randomized field trials, just in case-- RCTs are the way that they were known very early in the literature, and still nowadays in other disciplines. And then the non-experimental methods, all of this that you have here, some of which are in your get out the vote study.
All right. So before we go into what is a randomized experiment, I want to introduce the notion of validity. And Rachel raised it a little bit. But we usually think of in terms of two kinds of validity when you assess a study. The first one is internal validity. This has to do with your ability to draw causal inference. So your ability to attribute your impact estimates to the program. So if you said, this difference is my impact estimate, the study has strong internal validity if you can reliably attribute that to the program and not to something else for whatever population is represented in your study. So if you did the textbook project in Kenya, in a rural village in Kenya, well, that study-- if it's internally valid, or if it has strong internal validity, then it's going to be valid for the population represented by the sample you drew in Kenya, in that rural village in Kenya.
External validity, on the other hand, has to do with the ability to generalize to other populations, other settings, other time periods.
The reason I mention this is that these two things often trade off against each other when you are sort of trying to commission or conduct a study. So you may decide, I'm going to go this randomized trial in this very small place to test out my model. And you may be concerned with, how do I know if it generalizes to other settings? On the other hand, you may decide, well, I'm going to use other kinds of methods and be representative of the whole Kenya, or the whole India, or whatever country you're working in.
The key thing is to distinguish two things. The first one has to do with causal inference for your own sample, or for the population represented in your sample. The second one has to do with generalizability. And Rachel talked a little bit about how much you can generalize from experiments, and we can talk about that if you want.
All right. So what is a randomized evaluation? So the very basics-- can someone tell me what the basics are? Randomized experiments? How do you do it? How does it work?
There's one thing that you should know. When I first started teaching, I used to be very, very nervous when there was silence in the room. But now I'm very comfortable. So you tell me. So how does a randomized trial work?
AUDIENCE: Allocate the subject into the treatment of the control group based on a random assignment.
PROFESSOR: OK. random assignment. Sort of like a flip of a coin, right?
So in the simple scenario, we take a sample of program applicants-- just like we do with drug trials-- take a sample of program applicants and we randomly assign them either to a treatment group which is offered the treatment and a control group. They're not offered the treatment. This is a very simple setting, but the idea here is that by doing this, the treatment and the control group are comparable to each other. And so any differences you observe between these two groups should be attributable to the program.
The key about this method-- so this do not differ systematically at the outset of the experiment. The key about this method is that this control group is mimicking the counterfactuals. It's mimicking what will happen to the treatment in the absence of the treatment.
And the reason it's mimicking the counterfactual is that on average, this group should be exactly like the treatment group. So if we took all of you and we flip coins, from each of you we flip coins, and then you ended up in two different groups, the two groups would have, on average, the same characteristics. So the same people that come from a certain area of the world. The same percent of females. The same average intelligence. The same average income. The same average education. You name it. We're going to do an exercise where you can see this.
The beauty of this method is that the two groups statistically are going to be identical to each other. If they're not identical to each other statistically then you don't have random assignment. It has failed. Random assignment.
So the random assignment is the process you employ to create these two comparable groups. The huge advantage of this random assignment is that you don't need to think about, are the two groups the same on this characteristic that I care about? You don't need to think about that. The two groups should be the same on those characteristics.
AUDIENCE: So that's theoretically. So now thinking in terms of a program where you have, say, selection criteria. So let's say you want to do a program in a particular district, and you're looking for people that have three characteristics that are all the same. Let's say for whatever reason, the number of people that present themselves in that way is a relatively small number. Then you can randomly select within that small number. But then you're challenged by the size of your group.
PROFESSOR: Absolutely. And on Thursday, you'll get to that minimum sample size detected. But the key there, if those three characteristics are your selection criteria, you don't want to modify your selection criteria because someone is going to come and do an experiment. You want to offer the program to whoever you're going to offer the program. So those three characteristics are key for your program, because you decide those are the people you want to serve, then you need to find a way to do your evaluation that doesn't involve relaxing that criteria. Unless you really are thinking, well, it would be interesting to know if I served this other group, whether the program has a different effect or no.
AUDIENCE: But you can't mix and match among the criteria. You can't say-- or could you? Let's say you have trouble. You're not getting enough people with those three criteria. So you say, OK, now we're going to make it six criteria, and we'll be happy if they only meet four of the six. That right there would not make it possible to do this.
PROFESSOR: So if, at the end of your processes, where you're saying three criteria, six criteria, five, four, whatever you say-- if at the end of this process, you end up with a large enough pool to be able to randomly assign into two groups, treatment and control? No problem. You could have relaxed the criteria. You could have said six, five, four, whatever you want.
My previous answer is more to, don't change the criteria just because you want to do a randomized trial. You want to evaluate the program that you want to evaluate. You don't want to evaluate the program that you think will fit the randomized design. Make sense? Other questions, comments? No?
OK. So the two groups did not differ systematically at the outset of the experiment. I want to emphasize this. And again, there's going to be an exercise where you can see this in Excel. But the key is that the two groups will be identical both on observable characteristics and non-observable.
And when I say identical, they're identical statistically. It's not like the needs of these two groups are exactly the same. They are statistically identical in the sense that you should not observe a pattern of statistically significant differences between the two groups. If you were to test 100 characteristics, then five of them may end up being statistically significant, just because of the luck of the draw or multiple testing. But they shouldn't differ systematically at the outset of the experiment.
And this is the key. The whole key of impact evaluation is that then you can take that difference and attribute it to the program. And then you're not thinking, is it the program, or is it some pre-existing differences between the groups? If you reach the end of an impact evaluation and you're wondering, is it the program, or is it something else? Unfortunately, that's not a very good impact evaluation.
So there are some variations on the basics. You could assign to multiple treatment groups. So rather than having only one treatment, you could have multiple treatments. And this happens a lot if you're trying to test different ways of implementing a program. So you may have a program that you're thinking, well, I don't know of the best way to deliver it is method number one or method number two. And you may randomize into three groups. Method number one, method number two, and a control group. Or you may decide to do away with the control group and only randomize into, say, three methods, three ways of delivering an intervention. If you do away with the control group, you're going to be able to answer the question, is one treatment better than the other? But you're not going to be able to answer the question, is any of this treatment better than what would have happened in the absence of the program? So this is one variation.
And the other variation, we were talking about when Iqbal answered the question. He said, well, you have a bunch of people. You assign some to the treatment or to the control group. You can assign units other then people or households. Health centers, schools, local government, villages. And you can see in JPAL's website. There are a bunch of examples where each of these have been used as units for random assignment?
Yes? Your name, please? We don't have name tags, but I like to call people by their name. Wendy? Go ahead.
AUDIENCE: So if we pick schools, my conclusions will be about schools. They won't be about the students in the school. Or is that wrong?
PROFESSOR: So it depends on-- you say your conclusions will be about the schools? The key thing is, what is the unit of intervention here? So it's a program that's directed at all the children in the school, only some children in the school? In part, the decision of what you randomize, whether it's schools or children within schools, depends on what's the nature of the treatment.
So if you have a program that serves everyone in the school, yes. Your assignment should be at the school level. That is, you should have some schools that receive the program and others that don't. But if you have a program that is only going to serve some children in the school, then your assignment could be within the school, and you have some children who receive the treatment, and others that do not.
The key, though, is if you're using your second method, you want to make sure there are no spillovers. You want to make sure that someone receiving the treatment is not going to affect the outcomes of someone not receiving the treatment. And so you're going to see the spillovers. That's something you're going to see on Friday.
But the basic idea is, what level of randomization you have depends on, what is the level of your treatment? If you're treating schools, if you're treating individuals within schools, et cetera.
AUDIENCE: So statistically I want them to be the same.
PROFESSOR: You want them to be the same, yes.
AUDIENCE: My name is Manuel. Please talk a little bit about the unobserved characteristics.
PROFESSOR: Yes. So the unobserved characteristics-- this is something that a lot of the non-experimental methods wrestle with. And the idea is, the randomized experiment creates these two groups that, by pure laws of statistics, are identical in every single characteristic, statistically speaking. So both the ones you observe and the ones you don't observe.
So if we were trying to do an experiment in this classroom and I randomly assigned you into two groups, I can be confident that even things I don't observe about you, you're going to be balanced across those two groups. If instead I try to match you, I use all the information you gave me on your application forms and say OK, these people are from this-- I'm going to be able to do so with the observables, but not with the unobservables. And again, depending on how important these unobservable are in explaining the outcomes, that may be a big disadvantage or not so big disadvantage.
And this is what happened in the get out the vote example. You were able to observe some characteristics of people. And then non-experimental methods, all of them-- I mean, not all of them, but most of them-- can address those. Some of the methods can also address some unobservables, but again, they always rely on some assumption about how those unobservables behave. Here you're not relying on any assumptions. You need to do the random assignment properly, but once it's done properly, you're not relying on any assumption.
AUDIENCE: Is that the general dichotomy? There's randomized tests, and then matched pairs tests? Or is there other , is it generally broken down into those two?
PROFESSOR: So the way that I think most people break it down is randomized, where you use this random assignment, and then non-experimental methods. But I don't mean to imply that all the non-experimental methods are the same. And in fact, there are some people who called them quasi-experimental methods. Those people tend to think of them a little bit higher than the non-experimental methods. Non-experimental people tend to say, this is not good. Quasi-experimental, oh, this gets closer to the experiment.
But the key thing here is that whatever method you use, the key is how are the people getting into the program being selected, and how are you forming that comparison group, and what statistical techniques are you using to adjust for whether that comparison group is the same or not than the treatment?
So the dichotomy is not between randomized and matching. The dichotomy is usually between randomized and everything else. But within everything else, there are methods that are much better than others. Yes? [? Holgo? ?]
AUDIENCE: How do we randomize when we assign people into treatment and control groups, besides a lottery? [INAUDIBLE]
PROFESSOR: You mean the process? So tomorrow, the whole day is going to be about how to randomize. But the basic idea is, you can do it in a variety of ways. You can do it in a computer, which allows you a lot more flexibility. But if for any reason, you need to show people that you're doing it in a random, transparent manner, that can also be done.
We just did one in Niger in West Africa where we used bingo balls. So literally, people would draw from there, and then everyone could see. If we had brought a computer into their room in Niger and tried to do things, it just wouldn't have worked. People would have said, what are you doing here?
So there are there of many different ways of randomizing. The key-- and this is something we're going to talk about in a little bit-- is what exactly is the process that you use to make sure that it's random assignment, not the how, you know, whether it's bingo balls or a lottery or a coin or whatever it is. Yes?
AUDIENCE: So at what point this week will we talk about the ethical dimensions of denying treatment to someone?
PROFESSOR: OK. Like in three slides, you can jump at me with the ethical issues. And then if I don't satisfy you, you have four more days to jump at every single people who comes into this room.
So what I want to give you is a little bit of the nuts and bolts. Rather they keep this discussion in the abstract, this is what happens in the experiment. The nuts and bolts, if you wanted to do a randomized experiment tomorrow, these are sort of eight key steps that you need to think about.
This is a very simplified description of the process. As those people sitting in the back will tell you, this is very simplified. Their daily lives are consumed with many of the steps, and they work months, if not years, in each of this.
The first step, and I can't emphasize this enough, is to design the study carefully. So no matter what you do, what you do at the beginning is going to affect you study for the rest of the study. This is true for some things in life and not others. For evaluations, impact evaluations, if you don't do it right at the beginning, you're going to be in trouble. That's going to come down to haunt you. So anything you can do to spend time at the beginning, making sure that the study is designed properly, is going to be very helpful.
What that means, in very practical terms, is if you are in a position where you are commissioning a study, and you don't have people in your staff who are expert at this, make sure that whoever is going to help you do the evaluation is involved from the very beginning. What this also means is that calling someone three years after the program was implemented, saying, can you come and evaluate? That leaves the evaluator with very few options.
So the earlier the evaluators are involved, the better the options are in terms of how you can do this. Both in terms of the validity of the evaluation, but also in terms of how it will interact with the program in a way that it doesn't disrupt the program. So this is key.
And we can talk about design a little bit now, but you will learn a little bit about design when you speak about sample size, about measurement issues, and all of those sessions are coming. How to randomize. So Wednesday and Thursday are really about that.
The second one is to randomly assign people to treatment or control or more groups, if there are more than those.
The third one is to collect baseline data. So this is a big question that comes up. Should you collect baseline data? I think my answer to that is, in general, if you don't have a randomized evaluation, it's going to be very, very, very difficult to get away without baseline data. There are some methods that work, but it's going to be difficult. By baseline, I mean, before the intervention started.
If you have a randomized trial it would be highly preferable to have baseline data. Highly preferable. But not as critical as with other methods.
And it's preferable in two ways. The first one is if you have a baseline data, you can verify, at least in terms of those characteristics you collected in the baseline survey, you can verify that two groups look like. This is a nice thing to verify at the beginning and not at the end of the evaluation. So if you can do it, that would be helpful.
And the second thing you have to do is-- yes?
AUDIENCE: Sorry. What happens if, at the baseline data, you realize that the two groups that you made were not random? Do you go and keep randomizing until you get there?
PROFESSOR: So it depends. It depends on when you discovered this. If you discover this when the treatment is already being implemented, it is too late to do anything else in terms of re-randomizing. The ideal scenario is one in which you can do this, collect the baseline data, randomize, verify that they are similar, and then if they are not similar, then you can re-randomize again. There's controversy about how many times you should do this, but for the most part, in general, if you randomize, the two groups should look similar. There are very few scenarios, but they exist, where they don't look similar to each other. And if you reach one of those scenarios, you can re-randomize.
What you can't do is re-randomize when the treatment is already being distributed. So if you already decided, you're in the treatment group, you're in the control group, you can't re-randomize at that phase.
The second reason you want to collect data, and this is going to be important particularly in a setting like yours, if you are worried about sample size, is that it buys a lot of statistical power. Particularly if you can collect data on the baseline version of the outcomes that you care about. If you can do that, it's highly desirable. The reality is that sometimes it's feasible to collect baseline data and sometimes the nature of implementation of the program makes it difficult. But you will do well if you can collect baseline data.
AUDIENCE: Wouldn't it seem that by the very fact of collecting the baseline data, once we have already randomized, can bias this randomized by collecting the baseline data?
PROFESSOR: Because you're affecting the people who are answering the survey? Well, this has to do a little bit more with survey design than with any other thing. The key is, you're going to collect baseline data for both the participant or the treatment and the control group. So if you feel that when people answer a survey, they somehow-- I don't know-- get optimistic about life and do better or the other way around, as long as it happens in the same way for both treatment and control groups, it's not a problem for the randomized trials.
The problem would be if, for some reason, you think that administering a survey is going to affect the treatment and the control group differently. If that's the case, then you need to be careful about how you do the survey.
AUDIENCE: Can you explain how [INAUDIBLE] statistical power?
PROFESSOR: So in technical terms, what happens is, you, in your regression, where you estimate an impact, you have an outcome of interest. And that outcome has a variance, has some variations. And then if you can add into your regressions statistical controls, things you collected at baseline, what essentially happens is, in technical terms, the standard errors of your coefficients, particularly if these variables have a lot of explanatory power, those standard errors should drop, and you get more statistical power. Yes, Jessica?
AUDIENCE: Do you mean to say that you have to collect the baseline data after you do the first round of randomization? Does it matter what order you do those steps in?
PROFESSOR: Sorry. Steps two and three can be inverted. In fact, it would be ideal if you could invert them. It would be ideal, because then you can do what Iqbal is saying. Which is, you collect the baseline data, you do the randomization, and then you say, OK. Are they the same or not? Then if they're not the same, you re-randomize.
If you collect the baseline data after randomly assigning, unless you have not communicated to people who gets the treatment and who gets the control, your options for re-randomizing are not very good. So very good point.
All right. So the fourth step is to verify that the assignment looks random. By verifying that the assignment looks random, this is something that if you were to commission an evaluation, you should make sure that your evaluator provides to you this. Which is at the very least a table that says, here's the treatment group, here's the control group, and here's how they look like in terms of these baseline characteristics.
And ideally those two groups, those tables should have very, very few differences between the groups. When I say differences, they cannot be, in practical terms, large differences. There could be some differences that are statistically significant, because either you have a lot of statistical power, or more likely, if you compare 10 variables, some of them will end up being significant. The key is, there are no systematic differences between the groups. If you observe systematic differences, then you're in trouble. This didn't work well. But I can tell you from experience, from the law of statistics, these two groups will look the same a lot of the time.
OK. So obviously you can only do that verification if you have some data on the two groups before. Now, when I say "collect baseline data," if maybe you already have baseline data-- for some reason this is a population that you're ready serving, you already did surveys on these people-- if that's the case, then all the better.
It may be that you don't have baseline data, but you may be able to get baseline data. So for example, if you're randomly assigning schools, you may have, from the government or from some agency, some census of schools. And you may be able to compare schools in terms of socioeconomic characteristics of the students. You may be able to compare schools, you know, percent of private, public. If there was a test done nationally for all the schools, you may be able to compare test scores on those schools. The key thing is, anything you can do to verify that, will a random assignment work? Is good. It would be useful to do it at the beginning.
The fifth step is to monitor the process so that the integrity of the experiment is not compromised. This is something that's really, really key. When you do a randomized experiment, designing the study carefully is very important. Doing the random assignment is very important.
But you can't just relax and then wait for two years until you collect the outcomes. And the people who are sitting at the back of the room know this much better than I do. If you are not following exactly what's happening in the field, the opportunities for this experiment to not go well are very, very big. You're going to have a whole session on Friday on threats to an experiment. The only thing I will say now is that the best way to deal with threats to an experiment is to avoid those threats, and to avoid them at this stage of implementation.
One very quick threat. If you assign people to a treatment group and people to a control group, that means that people in the control group are not offered the treatment. But that also means, they shouldn't get the treatment. And as some of you know, that doesn't always happen. So some people in the control group find their way into the program. Having systems to monitor that this doesn't happen, and that if it does happen, that it happens in very, very few exceptional cases, is going to be very important. Yes, Logan?
AUDIENCE: One of the arguments for the superiority of the matched pairs is that if one treatment group ends up not getting the treatment because lack of capacity in that region, or vice versa, the scenario you described, you can just drop that pair.
PROFESSOR: Yes. The problem when you drop that pair is that it may be costly to you. Dropping that pair. And you have to assume that that-- well, first of all, you have to assume that pair was comparable to begin with. And then even if you were to drop that pair, well, first of all, matching doesn't always work on one-to-one. But even if you had one-to-one matching, suppose you had to drop 10% or 20% or 30% of your pairs, then you lose statistical power, and then you also lose external validity to begin with. Yes?
AUDIENCE: So there's also the issue of spillover effect, which isn't the same. So one might be that somebody sneaks into the program who was supposed to be in the program. But the other is, if you do things in the same community, which is often the case in the work that we do, or in a similar environment, the mere effect of having something going on--
PROFESSOR: Yes. And this is why the first stage is very important. If you think spillovers will occur, the moment to think about them is at the design stage of the evaluation. Because then you can decide on how you're going to randomize in a way that minimizes the effect that spillovers would have.
So there's some statistical techniques to deal with some of these problems. But the best way to do with these problems is to avoid them in the first place. And you avoid them by good design, where the evaluator can help, and by a good monitoring system to make sure that the evaluation is being implemented as intended. Makes sense?
Yes, your name please? Are you also filming from this camera here? OK. I'm nervous now. Two cameras.
AUDIENCE: What's on the [INAUDIBLE] to avoid [INAUDIBLE]?
PROFESSOR: Yes. So I think one important thing is to have people in the field who can help monitor, and who know about the evaluation. Two is to have a clear commitment. This is something that Rachel said this morning that's really, really key. Very clear commitment from whoever is organizing. That's very creative. For whoever is implementing the program.
So I'll give you an example. We were evaluating this program in Jamaica. And we were telling them, we need to monitor the crossovers. We can't have people who are not supposed to receive the program, get into the program. Yes, yes, yes. Is it OK if a few do it? We say, well, only if a few, but really, this has to be the exception, and you really have to monitor this rate, and we asked them for a report on this rate, and so on. This is a government agency in Jamaica.
And so they were all the time asking, OK. How many is too many? And we were like, no, no, no, no. You have to keep that rate to a minimum. There's no way you can have crossovers. Just keep-- no, but how many, how many? In one day of weakness, we said, OK. If it's more than 10%, this is completely ruined. We can't do anything with it.
So end of the evaluation arrived. We compute the crossover rate. 9.6%.
So what I want to say here is that if they didn't want to comply with our request, they could have made this rate be 30% or 40% and we would have not heard anything about it. I'm not saying 10% is the right threshold. It of course depends on the program and on other things.
But the key thing here is, you need to have full cooperation between the people in the field who are implementing and the people in the field who are evaluating. If you don't have that, then it's very difficult. Because people find a way to get to a program if they hear that this program is serving, is doing some good.
So I mean, who's a parent in this room? All right. So now, confess. If your child, in your school, there was a randomized trial on this very promising, you name it. After school program. And your child fell in the control group. Would you be at least tempted to go to the principal and say, I want my child in that program? Tempted? All right. I can tell you that other parents are more than tempted, and will find a way. All right.
AUDIENCE: What do you do with the spillovers? Do you just exclude them and put them in the comparison group?
PROFESSOR: So these are called crossovers, because they cross from the control to the treatment. The key thing-- this comes at the analysis stage, and this you'll do on Friday. But the key thing is, what random assignment buys you is that the two groups are comparable as a whole. The whole treatment group with the whole control group. You can't then just say, oh, I don't like this control group member. I'm just going to throw it out. That completely destroys the comparability. You still need to compare the full two groups, and you do some statistical adjustments to deal with the crossover.
But once a treatment, always a treatment. Once a control, always a control. The random assignment buys you that two groups are the same. If you throw away-- suppose then, 10% of crossovers. If you throw them away you will be comparing the whole treatment group with this 90% of the control group.
And let's just assume for a second that that 10% who crossover are people who are particularly motivated, and that's why they switch over. Well then, the average motivation of the two groups were the same at the beginning, but once you throw that 10% away, the average motivation of the treatment group is going to be higher than the average motivation of the control group. So any difference you find in outcomes between these two groups could be due to the program, but could also be due to differences in motivation. You can't throw them away. There's statistical ways of dealing with them. Yes?
AUDIENCE: Turns out, I guess I didn't understand the answer to the earlier question. So we're worried about spillover, and we're going to deliver books to-- clearly the intervention is that the kids get books that they can take home to study at night. But I've decided that because I'm worried about spillover and because it's more administratively convenient, I'm going to deliver to some schools. So I'm going to draw the schools at random, but I'm looking at the kids, impact on the kids.
PROFESSOR: That's OK.
AUDIENCE: So even so, I haven't damaged my ability to look at the students' effects, because my unit of randomization was at a different level.
PROFESSOR: That's perfectly fine. However, the higher the unit of randomization, the more trouble you're going to have in having enough statistical power to detect effects. But that's a topic that I want to leave up to Thursday.
But yes. I mean, when we say the schools are treated-- I mean, the schools are buildings. They're not being treated in any way. Unless you paint them or do something to them, they're not being treated--
AUDIENCE: Ours got paint.
PROFESSOR: OK. So if it's just painting them, then the schools-- no, but seriously. When I say treated, who's being affected by the treatment?
AUDIENCE: Well, I can't have a-- it's going to hurt my power. But I can randomize at a different level than [INAUDIBLE].
PROFESSOR: You can. Particularly if you want to avoid spillovers, that's exactly what you should be doing. All right. Yes?
AUDIENCE: My name is Cesar. What happened when the intervention is something about knowledge? For example, that some nurse trained to a treatment group about wash your hands, and this knowledge can--
PROFESSOR: Can spillover. Yeah. That's exactly right. So again, you need to think about the design of the study. If you really think it's going to spill over, then you need to think about randomizing at a higher level so that the spillover doesn't occur.
I do have to say one thing. There's some interventions where the spillover is evident. And you're going to see that in the deworming case. I think it's case number 4. So it's very clear that this is happening. There's a human biological transmission of disease that makes spillovers very clear.
This is my own bias. But there are tons of problem programs out there that have difficulty affecting the people that they're intended to. So thinking that they're going to affect other people they haven't been intending to help, in some cases at least, is a stretch.
Having said that, if you think spillovers will occur, then you need to think about that at the design stage of the study. yes? Your name please?
AUDIENCE: Yes, sir. Raj. Just getting back to the example where you were saying if you took each of us, and you assigned us to two different groups, it would adjust for the unobservable characteristics. Would that work out in a sample size so small?
PROFESSOR: In a sample size like this, you will have trouble with statistical-- I want to leave all those questions of-- you have our superstar, Esther Duflo, who's going to speak about statistical power. But the key thing here is, if you have a small group, then what happens is the sampling error is bigger. So you may observe differences between the groups. You may not declare them to be statistically significant because you have very little power.
So in general, you want larger sample sizes. This group is probably small. But even if you did it with this group, and I challenge you to do it-- just take an Excel spreadsheet and take five characteristics of you. And the random assignment, you're going to see some differences. But it's really amazing how the two groups will look alike.
And the other thing. If you're not accounting for unobservable differences like some non-experimental methods do. The key thing about this is, you don't need to account for anything, because the two groups are balanced across these two things. So they have the same average level of motivation, and so I don't need to control statistically for motivation. Because that cannot be a confounding factor if the two groups are the same. OK?
All right. So step number six. If you're going to measure the impact of a program on an outcome of interest, you need to collect data on that outcome. And that's called follow-up data. And the key thing is, you need to collect that for both treatment and control groups. And it's important that it be done in identical ways. So you can't, or it would not be a good idea, to have treatment group data come from one source, say, a survey, and control group data come from another source, say, administrative data, because data sources are generally not very compatible to each other.
The seventh step. Of course, estimate the program impact. And if the experiment is properly done, what you should be doing is just compare the outcomes-- the mean outcomes of the treatment group with the mean outcomes of the control groups. Now, there are versions of the experiments where they are more sophisticated, and then you need to use the multiple regression framework to control for things, particularly if you have stratified your sample, and so on.
But in general, the basic idea is, there are no differences between these two groups. Then the simple differences in outcomes between those groups should give you the impact of the program. There are other reasons you may want to use the regression framework, such as statistical power, that we were talking about before, but this is the basic idea. If the differences between the two groups is very different than what you get with the regression, you should start thinking about what's going on.
And then eight. And I think this is very important for practitioners. You should assess whether the program's impact are statistically significant, but also if they're practically significant. So if statistically significant means, we're confident that this impact is different from 0 in a statistical sense. Having said that, the impact may still be very small for any practical purposes. So it may be that a program affects some outcome of interest, but the effect is so small that you won't decide that this program was a success on the basis of that.
So both of those things are important. The stars or the asterisks for statistical significance are not enough for you to conclude that a program is successful. Yes? Your name pace.
AUDIENCE: Ashu. Yeah. I understand we can get the mean just by seeing the difference between the two sample sets. How do we get a handle on this trend of standard error and consequently the statistical significance?
PROFESSOR: Yeah. So again, in the simplest, very, very simple, you just do a comparison of two groups, this is the standard t-test, there's nothing else to do. In practice, a lot of this impact estimation is done through the regression framework. However you're going to do it, you're going to let your statistical software calculate those standard errors. Of course you need to be careful about things you learn on Thursday, such as clustering and so on. You need to make sure that those errors reflect that.
But the basic idea is, you let your statistical software or the evaluator calculate those impacts. But as a proxy, if the two means are not different, then it's going to be hard to argue that this program had a big effect.
OK. So random. As I said at the beginning, anyone can tell me, what does the term "random" mean? Yes?
AUDIENCE: Chosen by chance.
PROFESSOR: Oh, you work for public opinion polls. I should have asked you. All right. So "chosen by chance." What does that mean?
AUDIENCE: [INAUDIBLE] One can say random if there's no systematic trend behind the selection.
PROFESSOR: OK. Systematic trends. So you don't have someone saying, you go here you go there.
So suppose I wanted to do a random assignment in this classroom. And I went here, and I closed my eyes, and I throw a ball right here. I don't see where I'm throwing. I just throw it. Person gets it, falls into the treatment. Is that random?
AUDIENCE: No.
PROFESSOR: Why not? I already turned that way, right?
AUDIENCE: Maybe you like the sun.
PROFESSOR: Maybe I like the sun. And the people sitting near the sun may be different from the people who are not. Who knows.
The key thing is that when we say random, particularly in a simple randomized experiment, what we mean is that everyone, every single one of you, has the same probability of being selected into the treatment group. Or into one of the groups. Let's say the treatment group. So the key thing here is that Iqbal, Brook, Jamie, Jessica, everyone, Farah, everyone in this room, if we do a simple random assignment, you should have the same probability of being assigned to the treatment group.
So it has a precise statistical definition. It's not just someone saying, oh, yeah. We can't remember how we did it. It must have been random. No. It has a very, very precise definition. Because if you trust someone telling you, it was random, and then you trust that word, and then you start doing your study, and three years later, you discover it wasn't random, you are not going to be very happy with yourself.
So there are variations on this. If you have stratified, it doesn't mean that everyone must have the same probability. It means everyone within a strata. But the basic idea is, before we do random assignments, we should know the probability of everyone being selected. When I say the same probability of being selected into a treatment group, that probability doesn't need to be half. So it could be a third. It could be two thirds. From a statistical power perspective, you prefer half and half. But whatever it is, all of you should have the same probability of being selected. Make sense? OK.
AUDIENCE: In your example of drawing the ball, is that a random assignment?
PROFESSOR: Right. So again, it depends on the details on how you do it. But suppose we have balls for, I don't know, 30 participants or however many you are, and you have balls from 1 to 30, and you mix the bag, and you really trusted the physics that by mixing, that all the balls would have the same chance of being selected, and you draw one ball from the bag-- all the balls had the same chance of being selected. All of you had the same chance of being selected.
AUDIENCE: But the second person-- so when you draw one, that's 1 out of 30.
PROFESSOR: Yes.
AUDIENCE: But the second time you do it, you could have a--
PROFESSOR: So if the sample size is very, very small, you worry about sampling with replacement and without replacing-- if the population from which you're drawing is very small, you may have an issue with that. If the population is large, the difference between 1 in 1000 and 1 in 999, it's going to be pretty small. If you do it sequentially like that. If you do it in a computer, you can have a randomizing device that just generates a random number, and then you pick the first half.
OK. So is random assignment the same as random sampling? I see no, yes?
AUDIENCE: No.
PROFESSOR: No. I need a little bit more than that.
AUDIENCE: A random assignment, you would have already narrowed down to a smaller sample, and assigned within that sample. Random sampling would be taking a group out of a whole population.
PROFESSOR: OK. Very good. So one way think about this is you have your target population, then you have potential participants. This may be children you're targeting to in your intervention. And then you have your evaluation sample. Here's where the random sampling could occur. So-- sorry I forgot your name,
AUDIENCE: I didn't tell you.
PROFESSOR: You didn't tell me. This is even worse. Jean. So what Jean is saying is, random sampling happened at this stage. Or could have happened in this stage. What random sampling is buying you is the ability to generalize from your evaluation to this population here. And whether this is a population of policy interests or not, that's a different matter. But that's what random sampling is buying you.
What random assignment is doing is once you have the samples-- so suppose there are 100,000 potential participants. You don't have money to enroll 100,000 people in a program or in an evaluation. You pick, out of this 100,000, 5,000 at random, the results of your study are going to be generalizable to this 100,000. Now, within this 5,000, you do random assignment and you assign to a treatment group and to a control group. Maybe of this 5,000, 2,500 fall here, 2,500 fall here.
What random assignment buys you is these two groups are identical, and so any difference you observe in outcomes is due to the program. That's internal validity. That has to do with causal inference that is about this 5,000 that are here. So where the 5,000 generalize to is an external validation.
So they both have the word "random," but these are two different concepts. Again, random assignment relates to internal validity, causal inference. Random sampling refers to external validity. yes?
AUDIENCE: My name is Cornelia.
PROFESSOR: I should know it by now.
AUDIENCE: I haven't said it yet. Can you do one and not the other? Not really. Do you have to--?
PROFESSOR: You can, you can. In fact-- well, sorry. If it's called a randomized experiment, this one has to be there. This is what defines a randomized experiment. there was random assignment.
AUDIENCE: So you can do a randomized assignment, even if your sampling is not running.
PROFESSOR: That's right. So what that means is that then you need to think about who you generalize to.
All right. So advantages and limitations of experiments. For those of you who are a little bit more statistically inclined, the key thing about random assignment is that not only on average the two groups are the same, but the distribution, the statistical distribution of the two groups, is the same.
And this is very powerful for a lot of the adjustments that come at a later stage, particularly when there are crossovers and similar things. The idea is that the two groups not only on average both unobservable, and unobservable characteristics look the same, but the whole distribution. So they have the same variance, they have the same 25th percentile, the same 75th percentile. And of course, when I say the same, again, it's in a statistical sense, subject to sampling error, which we can account for. And so there are-- yes?
AUDIENCE: That doesn't necessarily mean that they're both anomolies.
PROFESSOR: No, no, no.
AUDIENCE: [INAUDIBLE]
PROFESSOR: Anything. Yeah. Anything. But the distribution should look the same.
OK. So no systematic differences between the two groups. This is deliberately a repeated slide. I didn't forget to take it out of the presentation. Key advantage, key takeaway message-- these two groups do not differ systematically at the outset, so any difference you observe should be attributable to the experiment. And this is under the big assumption that the experiment was properly designed and conducted. It's not like any experiment will reach this.
So other advantages of experiments. Relative to results from non-experimental studies, they're less subject to methodological debates. So a lot more boring conversations in academic seminars because there may be some questions about what question is being answered, there may be some questions about things that happen in the field that may have threatened the experiment. But the basic notion that if it was done properly, the two groups should look alike, it's never debated. Whereas with non-experimental methods, that's the whole sort of central claim of the seminar and of the presenter.
They're easier to convey. You can explain to people, look. These two groups look alike at the beginning. Now there's a difference. It must have been the program.
And they're more likely to be convincing to program funders and/or policymakers. If they find it more credible, easier to convey, it's more likely that they will take action. Although in this respect, I can't emphasize enough what Rachel said, which is, look. If you have the right question, then answering that question is going to be important to lead to change. If you have the wrong question, even if you did a nice experiment, it's not going to help you that much. Yes?
AUDIENCE: I've been to the conference two months ago. Some people were arguing that last first advantage that is with randomization-- that's random assignment-- how to build two groups that are identical to each other. And some people argue that you will almost never find a context where you will have that situation occur. The way the government programs operating in most cases, it is almost impossible that you find an exact identical treatment group and control group.
PROFESSOR: See, the key thing here is that you don't need to find it. It's not like you have a treatment group and now let's look in the whole country, where is the control group? No. This method forces the two groups to be the same. As long as there are some people who are going to be served by the program and some that are not, if you randomly assign to these two groups, the two groups should be identical. Not because you were very smart and looked for the other group, no. It's like random assignment is for those of us who precisely don't think we can come up with that other group on our own.
So there may be issues with whether you have enough program applicants to be able to divide them into two groups, participants and non-participants. But in context where you're not serving all the two groups-- so if you don't have money to serve 1,000 people, and 1,000 people applied to your program, and you only have 400 slots, that's not going to-- this goes to the ethical issue, which we'll discuss in a second. The only thing that changes is how you select those 400. But once you've selected randomly, those two groups should look identical.
Again, not because you were incredibly astute at saying, oh, here's another group. No. This this happens through the flip of a coin. This is not a researcher a kind of, oh, can the research and find a group? Or the context is development versus a developed country. This has to do with the technique applied to any setting.
Again, you're going to have a case where you see a spreadsheet and you can see, you can do the random assignment and see for yourself that the two groups will look similar. OK?
AUDIENCE: Is it necessary that the size of the two groups have to be the same?
PROFESSOR: No, it's not necessary. And in fact in practice, what happens is, suppose you had 1,000 applicants and you had money to serve 600. Then no matter what the statistician says-- oh, it would be nice to have 500 and 500-- you're not going to have 100 people not being served just because you want to keep the half-half ratio.
From a statistical perspective it's ideal to have 50-50 ratio, but only from a statistical prospective. If you deviate too much from that 50-50, then you get in trouble. So if you get to-- I don't know. The rule of thumb may be different for different people. But if you get over 70-30, I would say probably you're going to lose a lot of statistical power by doing that.
AUDIENCE: Yeah, but in some cases, for example, a country needs to make priority in aid with about 200 hospitals, for example. And in my country, there are one hospital that is the most important public hospital in Honduras. So you can apply this randomized process. But if you don't include this particular hospital, you cannot include this particular hospital because it's too important. We call that [UNINTELLIGIBLE] [? represented ?] subject for this type of problem, who have the possibility of 1. Should be in the sample. I don't know if you understand my Spanglish.
PROFESSOR: No, no. I speak Spanish. We can communicate here. So the key thing is, Again, you're trying to create comparable groups. If for some reason you need to serve a hospital because the president of your country says, you need to serve this hospital, that's fine. One slot. But that hospital should not be a part of your study, because that hospital was not randomly assigned. That's all. As simple as that.
And you may have a few of those. I mean, I can tell you, in my own experience, we're trying to implement random assignment in Niger, a program financed by the Millennium Challenge Corporation. A program about building schools. We said, we're going to do a random assignment. And they say, yes, yes, yes. Well, the US ambassador visited two of the villages, and he promised them they were getting schools. Now, you tell me if you want to be the evaluator and tell those schools, no, no. We're going to put you in the pool of-- no way. Those two villages are going to get their schools, but they're not part of our evaluation.
AUDIENCE: Is there an acceptable margin?
PROFESSOR: See, that's again the Jamaica question. I won't make that mistake again. I won't to tell you.
You're going to see on Thursday a whole session on statistical power, and you're going to get a sense of where you are. You don't want to have too many first, because you lose sample size, and second because you lose representativeness. I mean, in the case of the hospital in Honduras, if that's the hospital where 90% of things are happening, then it's a little bit hard to have that as a hospital that's out of your study. So that is an important issue.
All right. There are limitations of experiments, believe it or not. So the first one is, huge methodological advantages. But you still need to worry about these issues of internal validity and external validity. And what I would say about this is, on Friday youo're going to learn a lot about how to do with these internal validity issues. And I'm not going to go over them now. But the key thing is, if you can avoid them from the beginning in terms of how you design your program and how you implement them, then much better.
External validity issues-- as Rachel said, any impact evaluation conducted in a particular setting is going to have external validity issues. But experiments are particularly prone to them because they're sometimes done in particularly concentrated areas where you really want to find out, does this program work before expanding it, so the external validity issue is there. As Rachel said, if you can design an experiment to test each thing in your theory of change, that usually helps with external validity. And of course, if you can replicate evaluation in other settings.
AUDIENCE: So OK, you're going to have 10 variables with internal validity, equal internal validity, but only three variables with external validity?
PROFESSOR: When you say three variables, what do you mean with variables?
AUDIENCE: The variables that you are--variables. The study variables. I mean, when you're going to evaluate internal validity, you're going to have 10 variables or 20.
PROFESSOR: Well, internal validity, the two groups should be the same. And you have pretty strong internal validity if you can deal with this problem.
AUDIENCE: When you're going to the external validity, maybe not the whole 20 variables will have external validity. But maybe your three or four where you have been made different experiment in--
PROFESSOR: So it really depends on the context of your project. Again, I think the good example is deworming. So deworming, you take out worms. Well, in Honduras, if children who go to school, there are no worms, and that's not the reason they don't go to school, then that program in Kenya doesn't have much external validity or generalizability to Honduras.
So you need to be thinking about how the effect is supposed to be happening. And here there was the anemia thing, which may work in the case of Honduras or not. You need to be seeing, what is the chain? And seeing whether that chain is likely to hold in whatever other contexts you want to apply. There's no magic formula here.
AUDIENCE: Yeah, but you are going to control the theoretical framework with just three, four variables because that variable will be common in different countries?
PROFESSOR: Yeah, but you can have 200 variables. You can say, it depends on so many things. But there's a limit to how much-- the external validity issue is an issue that you can always hide behind it. You can always say, oh, this program worked in Kenya. Who knows whether it would work somewhere else?
And then if you take that attitude, then you can't learn anything from a randomized experiment, or from any impact evaluation that's done in a specific setting. Because even if you did it in Kenya, in a particular point in time, you can always say, well, it worked in Kenya ten years ago, but maybe it won't work today.
So I lean to the middle ground here. You sort of think about what are the critical steps or stages in which it can work, and then go implement it, and maybe evaluate it. I think my answer here is, external validity issues are going to be present for both experiments and non-experiments. There is no magic formula here. As long as you evaluate in a particular setting, you're still going to be subject to the question, does it work in some other setting?
Some of these threats also affect the validity of non-experimental studies. The key thing, though, is that some of this, in the non-experimental studies, you may not even realize that you have the threat. Because you've already done something that allows you to be blind to the threat.
So other limitations, the experiment measures the impact of the offer of the treatment. So when we implement the program, and we say, OK, you are in the treatment group, you're going to get the program, as you know from implementing these programs in the field, not all of the people you offer the program are going to take up the program.
So what the experiment buys you is, the whole treatment group is comparable to the whole control group. So the experiment is going to tell you, this is the impact for every, on average, for the whole treatment group. So some of them may not have received the program, and some of them may be diluting the impact of the program when you estimate. But technically, that's the impact that the experiment is estimating. So if you have a program with a very low take-up rate, then you need to worry about the issue that the non-takers are going to dilute the effect of the program.
You can then go and calculate, what is the effect of the program for those who participated? But then you start relying on non-experimental assumptions. You've lost a bit the advantage of the experiment. So that's something that you need to think about when you do an experiment.
There's a limitation in terms of these experiments can be costly. I'll sort of just say two things about being costly. I'll say three things about being costly. And I did learn that I should never say "I'll say three things," and I'll forget what those three things are. But I think I'll keep them in mind.
The first thing-- a lot of the cost of an experiment is data collection. So if you are trying to evaluate the impact of a program through some other non-experimental method that involves data collection, you've already made the two costs pretty comparable. Because again, data collection is a big cost. If you had a non-experimental method where you don't have to collect data, obviously there's no question that that is going to be cheaper. So it can be costly. But again, main cost data collection, which may be the same for non-experimental studies that collect data.
But the other thing about the experiment in terms of cost is that the same sample size buys you more statistical power. And you may see some of this on Thursday. So if you have a sample size of 1,000 people for an experimental study and a sample size of 1,000 people for a non-experimental study, those data collections' cost will be identical, but they will be buying you different statistical power. So that's one thing to keep in mind about the cost of experiments.
And the last thing is, you need to factor in, what is the cost of getting the wrong answers? If you really think that non-experimental methods are not going to work in your particular context, then it's not so useful to invest less money if you don't think you're going to get the same answer.
And again, I don't want to push the notion that only with an experiment you'll get the right answer. But if you think with a non-experiment, you won't get the right answer, then the cost of the wrong answer, the risk of a wrong answer.
Ethical issues. Throw them at me.
AUDIENCE: How do you say no to people who come to you, saying I want to put myself in this program. I have all the characteristics you're asking for. You're offering it to my neighbor. How come you're not offering it to me?
PROFESSOR: OK. The first thing to think about here is experiments are typically done in context where there's access demand. Where there are more people who want to be in your program than can be served by your program. And if that's the case, suppose you had 1,000 people who applied to your program, and you can only serve 400.
The question I ask you, Cornelia-- and only you-- is how many people are you going to have to say, sorry, I can't serve you? 600. Both in the context of an experiment and in the context of a non-experimental study. The only thing that changes is how you decide who those 600 people are. It's the only thing that changes. And in fact, in some contexts, the flip of the coin can seem more fair then you deciding, I think this person is more deserving, or this person--
So in that context, in the context where you're going to have to turn away people, then the ethical issues, in my mind, are much harder to justify. I'm not saying there are no ethical issues in experiments. There are some context in which there are ethical issues.
So if you are completely convinced that your program works, then why are you going to do this whole randomized experiment? The only thing I can tell you is that a lot of people have been very convinced that some programs work, and then they turn out not to work. But if you are completely convinced that the program works, then you shouldn't be doing it.
And then the other thing is, if you are testing an intervention that you think can harm people, then there are ethical issues involved. So I don't think anyone will be very fond of doing an experiment to try to find out whether smoking causes lung cancer, for example. Because we don't have experimental evidence, but the medical evidence seems to be pretty strongly in favor of that. Maria Teresa?
AUDIENCE: A consequence of that ethical question, was hard for me, was people who are indeed chosen to be in the program and people who are not. You have to come back to these people who are not and follow up with them. And how willing to cooperate were they to collect more data, to talk with them. And you know, working [UNINTELLIGIBLE] is really hard, because you take time from the farmer for two hours every couple months, and come back, and standing there. I mean, while the other guy received something for these two hours that are given to you. So I think that that is the-- Maybe you need to apply this more often.
PROFESSOR: Yeah. So I mean, again, I think there are things you try to do to deal with them. That has to do more with the implementation of any study in which you have a comparison group. It's not the experiment. Experiment has a control group. With any other study that has a comparison group where you're collecting data faces this issue.
And then there are things you can do. It depends on the program. But certainly sometimes offering some small incentive for people in both groups to fill in the survey is certainly one thing that could help.
The other thing that I think is very important is data collection. The average researcher, when they are asked the question, do you want to add one more question to the survey? The probability of saying yes is 99% for the average researcher. So if you have two hours in the field, you have to start thinking, well, how many of this question do I really need to be asking? I mean, that's an issue of implementation versus--
So I think there ways to do with this. But again, it's not unique to experiment. It really has to do with how you implement any study in which you're going to collect data on people who are not receiving any benefit. Yes? Ethical issues?
AUDIENCE: Nigel. I think an answer which--
PROFESSOR: Nigel. You are from the Kennedy School. Very nice to meet you.
AUDIENCE: I'm leaving next week. The issue of, even if you had as much money as you kept to all give to those 1,000 people, you can't do them all today. So the way to do it is say, OK, we'll do 500 this year and 500 next year. So you're getting all 1,000 people, but you do your randomized evaluation year one.
PROFESSOR: Exactly. And tomorrow there are going to be two sessions on how to do roll out design-- there's a bunch of designs that are applying the same principle.
AUDIENCE: When you think about the cost of the study, don't you think a question you should deal with way early on is the size of the impact that you're looking for?
PROFESSOR: Absolutely.
AUDIENCE: If the study is going to cost me a lot of money, and there's a significant probability that it might have only a small effect, then that maybe isn't worth bothering with. And so you talked about looking up the size of the effect and the statistics, and whether it's statistically significant. But that size question, it seems to me, gets looked at very late. And it should be way up front in the very early days because of the impact, whether the program is really of interest, and worth following.
PROFESSOR: So two quick reactions. The first one is what Rachel said. Think strategically about impact evaluations. You don't want to evaluate every single thing that's in your organization or every single thing under the sun. You're not going to be able to do an impact evaluation on all of those things. You may do other kinds of evaluations on hopefully most of your programs, but an impact evaluation, you should be very strategic on where you do it. And if you think this is a program that is not generating much impact and it's not costing you that much money, then you may say, I'm not going to evaluate it.
The second thing I would say with regard to that is thinking about the effect of the program is something you need to do at stage one, the designing of the study. And this will connect with your session on sample size that Esther will speak about on Thursday. Because thinking about the larger that impact is, that affects your calculations of sample size.
The paradox in all of this, despite of what you said, the paradox in all of this is that the bigger the effect of the program-- so if you expect this program is going to have a huge effect-- the smaller the sample size you need, and hence the smaller the data collection costs. So paradoxically, if the program is extremely important, the data collection cost should actually be lower than a program where you want to detect effects that are very small.
Having said that, you want to evaluate the programs that make strategic sense for you to evaluate. I mean, one thing I think you should try to avoid, despite all our enthusiasm with randomized experiment, you shouldn't leave this course thinking, OK. Where do I see an opportunity to randomize? And then forget about what is it that you're trying to do. You know, you may find a great opportunity to randomize, but if it doesn't answer a question you care about, you've just wasted money.
All right, so-- you have a question? This is very interesting.
AUDIENCE: I want to know, do you think that in any context, one can be able to carry out an impact evaluation? For any type of program--
PROFESSOR: So my answer to that is no, not in any context. But probably in more contexts than you think about. That is my short answer.
AUDIENCE: What about, for example, infrastructure--?
PROFESSOR: There have been. It's harder to do. There have been some studies. This is actually, I think, a growing area. This is an area where people are trying to do some impact evaluation. I mean, if you're building a road in the middle of the country, and this is one road for the whole country-- you can't do it.
But it's OK. You don't need to do an impact evaluation for everything you do, and you don't need to do a randomized impact evaluation for everything you do. What I do hope the message comes clear is, if you decide to do an impact evaluation, then thinking about a randomized design should be your first choice. If you can't do it-- and can't do it is not just, oh, there's some issues-- no, no. Can't do it, really trying, given all these advantages, really trying-- if you can't do it, then you may consider doing other things. But this should be your first option if you decide to do an impact evaluation.
All right. Partial equilibrium. It's a little bit more technical. But if you have a program that only affects some people differentially. So suppose you had a program that was going to train people on how to have better resumes. And if you only do it for a few people, then this program may have a huge effect. But if you do it for everyone in your town, there's going to be little advantage that's gained from this. And so the randomized experiment estimates a partial equilibrium effect. You don't know what would happen if everyone in a particular setting got the treatment. I think this is important in some settings, but not enough.
All right. So I'm not going to go too much about get out the vote, because we're already a minute away from time. What I want to do is just show you this table here. You already discussed it.
So this is what the case study shows. This is a situation where you had four methods to estimate impacts. The first four methods found out that the program had an effect. The last method, the randomized experiment, found no statistically significant effect. I'm not saying that in every single-- this goes back to your question. I'm not saying that in every single setting, this will happen. But this is a good example of a setting in which if you had gone with any of these techniques, you would have concluded the program had an effect when it didn't. And there are other settings where the reverse may happen.
And so if we were able to say ex ante, before the evaluation, this method is going to be just as good as the experiment, that's great. We may be able to save some money if there's no data collection involved, and that would be great. But I think the bottom line here is, we are not always able-- and I think very few people will tell you, we know when this method will work. Because the assumption behind each of this methods on how the work is untestable-- you can't statistically test that assumption. So you may argue in favor of it. You may show evidence in favor of it. But you can't specifically test it. And that's the big advantage of the experiment.
So let me just close with what I hope are the bottom lines from this. The first thing, what's underlined there. If properly designed and conducted, the social experiments provide the most credible assessment of the program. But the "if" is a very important "if." Don't leave this course thinking, if it's a randomized experiment, piece of cake. Everything will work. That's not the message that we want to give you here. It needs to be properly designed and conducted. And for that, you really need a partnership between the evaluators and the agencies implementing it. They're easy to understand, much less subject to the methodological quibbles, and more likely to convince policymakers.
These advantages are only present if they are properly conducted and implemented, and you must assess the validity of experiment in the same way you assess the validity of any studies. Because you're going to have threats to an experiment anyway, and on Friday, you're going to learn how to deal with some of them.
I hope this was moderately helpful. I think I have one of the toughest sessions to teach, because you guys, some of you come completely convinced of why you want to randomize, some of you come very skeptical, and I have to reach a middle ground. I hope I did. If you have one more question, I'll take it. Yes?
AUDIENCE: Have you found that it's possible to teach organizations to run their own randomized trials from start to finish, even if there are no economists on staff? Or does this always sort of require the intervention or assistance of outside modulators?
PROFESSOR: I think, as you will see throughout this course, conducting an impact evaluation, even a randomized one, does involve some technical skills and does involve some practical experience in doing it. I'm not saying those cannot be found in organizations that are in the field. But if those skills are not there, it's going to be very hard to do it.
Now, you can do a lot of training on how to do this things. But I think it'd be hard to do it without someone who has at least done a few of these and seen some of the problems that arise. Because problems will arise-- I mean, no question about it. You will be asking the evaluator, how far can we go? And the evaluator, whoever it is, whether they're in the agency or not, needs to be able to answer that question in a way that at the end, you have a credible evaluation.
I'm not saying you need an expert outside of the organization. But I am saying you need an expert somewhere. And whether you have it inside or outside, there's a whole issue of independence versus objectivity that I won't speak to.
AUDIENCE: Consumer companies do it.
PROFESSOR: Consumer companies?
AUDIENCE: Yeah. Procter & Gamble and big companies like that do experiments all the time, build their capability into the organization, how they make decisions.
I'm just wondering that if someone leaving this course with a few experiments under their belt could implement something like this, or whether you need to go as far as getting an economics degree in order to be able to do the coordinating and evaluation of this type.
PROFESSOR: So I think to do an impact evaluation, there are usually more than one people involved. And there are different roles for different people. There are some roles who are having good training in economics as particularly useful. There are other roles where I would say it's particularly un-useful to be an economist.
So I really think it depends on what role a person leaving this course would like to sort of play in the evaluation. And you know, whether leaving this course, you'll be able to run your experiments on your own-- I think would be an extremely successful course if that happened. We have no way to measure the impact of this program, but if that were to happen, relative to what would have happened if you had not come to this course, that would be phenomenal.
I think my sense is unless you have prior training in this kind of thing, what this course will hopefully give you is the ability to be involved in an evaluation and to be pretty good at interacting with whoever is also involved in evaluation at asking the right question of the evaluator. This is extremely important. And being very aware in the field of what may be threatening an evaluation. If you're able to do it on your own after this, I hate to say it, but I don't think it's because this session that you heard from me today.
All right. I think I already ate a few minutes into your time. It was a pleasure. I'll be here for a few more minutes if you want. I hope you have a wonderful rest of the course, and see you somewhere.