Flash and JavaScript are required for this feature.
Download the video from iTunes U or the Internet Archive.
Topics covered: Advanced Topics
Instructors: Prof. Erik Demaine, Prof. Charles Leiserson
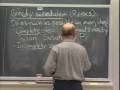
Lecture 22: Advanced Topics
Related Resources
We only have four more lectures left, and what Professor Demaine and I have decided to do is give two series of lectures on sort of advanced topics. So, today at Wednesday we're going to talk about parallel algorithms, algorithms where you have more than one processor whacking away on your problem.
And this is a very hot topic right now because all of the chip manufacturers are now producing so-called multicore processors where you have more than one processor per chip.
So, knowing something about that is good.
The second topic we're going to cover is going to be caching, and how you design algorithms for systems with cache.
Right now, we've sort of program to everything as if it were just a single level of memory, and for some problems that's not an entirely realistic model.
You'd like to have some model for how the caching hierarchy works, and how you can take advantage of that.
And there's been a lot of research in that area as well.
So, both of those actually turn out to be my area of research.
So, this is actually fun for me.
Actually, most of it's fun anyway.
So, today we'll talk about parallel algorithms.
And the particular topic, it turns out that there are lots of models for parallel algorithms, and for parallelism.
And it's one of the reasons that, whereas for serial algorithms, most people sort of have this basic model that we've been using. It's sometimes called a random access machine model, which is what we've been using to analyze things, whereas in the parallel space, there's just a huge number of models, and there is no general agreement on what is the best model because there are different machines that are made with different configurations, etc. and people haven't, sort of, agreed on, even how parallel machines should be organized. So, we're going to deal with a particular model, which goes under the rubric of dynamic multithreading, which is appropriate for the multicore machines that are now being built for shared memory programming. It's not appropriate for what's called distributed memory programs particularly because the processors are able to access things.
And for those, you need more involved models.
And so, let me start just by giving an example of how one would write something. I'm going to give you a program for calculating the nth Fibonacci number in this model.
This is actually a really bad algorithm that I'm going to give you because it's going to be the exponential time algorithm, whereas we know from week one or two that you can calculate the nth Fibonacci number and how much time?
Log n time. So, this is too exponentials off what you should be able to get, OK, two exponentials off.
OK, so here's the code.
OK, so this is essentially the pseudocode we would write.
And let me just explain a little bit about, we have a couple of key words here we haven't seen before: in particular, spawn and sync.
OK, so spawn, this basically says that the subroutine that you're calling, you use it as a keyword before a subroutine, that it can execute at the same time as its parent. So, here, what we say x equals spawn of n minus one, we immediately go onto the next statement. And now, while we're executing fib of n minus one, we can also be executing, now, this statement which itself will spawn something off.
OK, and we continue, and then we hit the sync statement. And, what sync says is, wait until all children are done.
OK, so it says once you get to this point, you've got to wait until everything here has completed before you execute the x plus y because otherwise you're going to try to execute the calculation of x plus y without having computed it yet.
OK, so that's the basic structure.
What this describes, notice in here we never said how many processors or anything we are running on.
OK, so this actually is just describing logical parallelism -- -- not the actual parallelism when we execute it.
And so, what we need is a scheduler, OK, to determine how to map this dynamically, unfolding execution onto whatever processors you have available.
OK, and so, today actually we're going to talk mostly about scheduling. OK, and then, next time we're going to talk about specific application algorithms, and how you analyze them.
OK, so you can view the actual multithreaded computation.
If you take a look at the parallel instruction stream, it's just a directed acyclic graph, OK?
So, let me show you how that works.
So, normally when we have an instruction stream, I look at each instruction being executed.
If I'm in a loop, I'm not looking at it as a loop. I'm just looking at the sequence of instructions that actually executed.
I can do that just as a chain. Before I execute one instruction, I have to execute the one before it.
Before I execute that, I've got to execute the one before it. At least, that's the abstraction. If you've studied processors, you know that there are a lot of tricks there in figuring out instruction level parallelism, and how you can actually make that serial instruction stream actually execute in parallel.
But what we are going to be mostly talking about is the logical parallelism here, and what we can do in that context. So, in this DAG, the vertices are threads, which are maximal sequences of instructions not containing -- -- parallel control. And by parallel control, I just mean spawn, sync, and return from a spawned procedure. So, let's just mark the, so the vertices are threads. So, let's just mark what the vertices are here, OK, what the threads are here.
So, when we enter the function here, we basically execute up to the point where, basically, here, let's call that thread A where we are just doing a sequential execution up to either returning or starting to do the spawn, fib of n minus one. So actually, thread A would include the calculation of n minus one right up to the point where you actually make the subroutine jump. That's thread A.
Thread B would be the stuff that you would do, executing from fib of, sorry, B would be from the, right. We'd go up to the spawn.
So, we've done the spawn. I'm really looking at this.
So, B would be up to the spawn of y.
OK, spawn of fib of n minus two to compute y, and then we'd have essentially an empty thread.
So, I'll ignore that for now, but really then we have after the sync up to the point that we get to the return of x plus y.
So basically, we're just looking at maximal sequences of instructions that are all serial.
And every time I do a parallel instruction, OK, spawn or a sync, or return from it, that terminates the current thread.
OK, so we can look at that as a bunch of small threads.
So those of you who are familiar with threads from Java threads, or POSIX threads, OK, so-called P threads, those are sort of heavyweight static threads.
This is a much lighter weight notion of thread, OK, that we are using in this model.
OK, so these are the vertices. And now, let me map out a little bit how this works, so we can where the edges come from. So, let's imagine we're executing fib of four. So, I'm going to draw a horizontal oval. That's going to correspond to the procedure execution. And, in this procedure, there are essentially three threads.
We start out with A, so this is our initial thread is this guy here. And then, when he executes a spawn, OK, he's going to execute a spawn, we are going to create a new procedure, and he's going to execute a new A recursively within that procedure.
But at the same time, we're also going to be, now, aloud to go on and execute B in the parent, we have parallelism here when I do a spawn.
OK, and so there's an edge here.
This edge we are going to call a spawn edge, and this is called a continuation edge because it's just simply continuing the procedure execution.
OK, now at this point, this guy, we now have two things that can execute at the same time.
Once I've executed A, I now have two things that can execute. OK, so this one, for example, may spawn another thread here.
Oh, so this is fib of three, right?
And this is now fib of two. OK, so he spawns another guy here, and simultaneously, he can go on and execute B here, OK, with a continued edge. And B, in fact, can also spawn at this point. OK, and this is now fib of two also. And now, at this point, we can't execute C yet here even though I've spawned things off. And the reason is because C won't execute until we've executed the sync statement, which can't occur until A and B have both been executed, OK? So, he just sort of sits there waiting, OK, and a scheduler can't try to schedule him.
Or if he does, then nothing's going to happen here, OK? So, we can go on.
Let's see, here we could call fib of one.
The fib of one is only going to execute an A statement here.
OK, of course it can't continue here because A is the only thing, when I execute fib of one, if we look at the code, it never executes B or C. OK, and similarly here, this guy here to do fib of one. OK, and this guy, I guess, could execute A here of fib of one.
OK, and maybe now this guy calls his another fib of one, and this guy does another one. This is going to be fib of zero, right? I keep drawing that arrow to the wrong place, OK?
And now, once these guys return, well, let's say these guys return here, I can now execute C.
But I can't execute with them until both of these guys are done, and that guy is done. So, you see that we get a synchronization point here before executing C.
And then, similarly here, now that we've executed this and this, we can now execute this guy here.
And so, those returns go to there.
Likewise here, this guy can now execute his C, and now once both of those are done, we can execute this guy here. And then we are done.
This is our final thread. So, I should have labeled also that when I get one of these guys here, that's a return edge.
So, the three types of edges are spawn, return, and continuation. OK, and by describing it in this way, I essentially get a DAG that unfolds.
So, rather than having just a serial execution trace, I get something where I have still some serial dependencies.
There are still some things that have to be done before other things, but there are also things that can be done at the same time. So how are we doing?
Yeah, question? Is every spawn were covered by a sync, effectively, yeah, yeah, effectively.
There's actually a null thread that gets executed in there, which I hadn't bothered to show.
But yes, basically you would then not have any parallelism, OK, because you would spawn it off, but then you're not doing anything in the parent. So it's pretty much the same, yeah, as if it had executed serially.
Yep, OK, so you can see that basically what we had here in some sense is a DAG embedded in a tree.
OK, so you have a tree that's sort of the procedure structure, but in their you have a DAG, and that DAG can actually get to be pretty complicated. OK, now what I want to do is now that we understand that we've got an underlying DAG, I want to switch to trying to study the performance attributes of a particular DAG execution, so looking at performance measures.
So, the notation that we'll use is we'll let T_P be the running time of whatever our computation is on P processors.
OK, so, T_P is, how long does it take to execute this on P processors? Now, in general, this is not going to be just a particular number, OK, because I can have different scheduling disciplines would lead me to get numbers for T_P, OK?
But when we talk about the running time, we'll still sort of use this notation, and I'll try to be careful as we go through to make sure that there's no confusion about what that means in context.
There are a couple of them, though, which are fairly well defined. One is based on this.
One is T_1. So, T_1 is the running time on one processor. OK, so if I were to execute this on one processor, you can imagine it's just as if I had just gotten rid of the spawn, and syncs, and everything, and just executed it.
That will give me a particular running time.
We call that running time on one processor the work.
It's essentially the serial time.
OK, so when we talk about the work of a computation, we just been essentially a serial running time.
OK, the other measure that ends up being interesting is what we call T infinity. OK, and this is the critical pathlength, OK, which is essentially the longest path in the DAG. So, for example, if we look at the fib of four in this example, it has T of one equal to, so let's assume we have unit time threads. I know they're not unit time, but let's just imagine, for the purposes of understanding this, that every thread costs me one unit of time to execute. What would be the work of this particular computation? 17, right, OK, because all we do is just add up three, six, nine, 12, 13, 14, 15, 16, 17.
So, the work is 17 in this case if it were unit time threads.
In general, you would add up how many instructions or whatever were in there. OK, and then T infinity is the longest path. So, this is the longest sequence. It's like, if you had an infinite number of processors, you still can't just do everything at once because some things have to come before other things. But if you had an infinite number of processors, as many processors as you want, what's the fastest you could possibly execute this?
A little trickier. Seven?
So, what's your seven? So, one, two, three, four, five, six, seven, eight, yeah, eight is the longest path.
So, the work and the critical path length, as we'll see, are key attributes of any computation.
And abstractly, and this is just for [the notes?], if they're unit time threads.
OK, so we can use these two measures to derive lower bounds on T_P for P that fall between one and infinity, OK?
OK, so the first lower bound we can derive is that T_P has got to be at least T_1 over P. OK, so why is that a lower bound? Yeah?
But if I have P processors, and, OK, and why would I have this lower bound? OK, yeah, you've got the right idea. So, but can we be a little bit more articulate about it? So, that's right, so you want to use all of processors.
If you could use all of processors, why couldn't I use all the processors, though, and have T_P be less than this? Why does it have to be at least as big as T_1 over P? I'm just asking for a little more precision in the answer. You've got exactly the right idea, but I need a little more precision if we're going to persuade the rest of the class that this is the lower bound.
Yeah?
Yeah, that's another way of looking at it.
If you were to serialize the computation, OK, so whatever things you execute on each step, you do P of them, and so if you serialized it, somehow then it would take you P steps to execute one step of a P way, a machine with P processors.
So then, OK, yeah?
OK, maybe a little more precise.
David?
Yeah, good, so let me just state this a little bit.
So, P processors, so what are we relying on?
P processors can do, at most, P work in one step, right? So, in one step they do, at most P work. They can't do more than P work.
And so, if they can do, at most P work in one step, then if the number of steps was, in fact, less than T_1 over P, they would be able to do more than T_1 work in P steps. And, there's only T_1 work to be done. OK, I just stated that almost as badly as all the responses I got.
[LAUGHTER] OK, P processors can do, at most, P work in one step, right?
So, if there's T_1 work to be done, the number of steps is going to be at least T_1 over P, OK?
There we go. OK, it wasn't that hard.
It's just like, I've got a certain amount of, I've got T_1 work to do. I can knock off, at most, P on every step. How many steps?
Just divide. OK, so it's going to have to be at least that amount. OK, good.
The other lower bound is T_P is greater than or equal to T infinity. Somebody explain to me why that might be true. Yeah?
Yeah, if you have an infinite number of processors, you have P. so if you could do it in a certain amount of time with P, you can certainly do it in that time with an infinite number of processors.
OK, this is in this model where, you know, there is lots of stuff that this model doesn't model like communication costs and interference, and all sorts of things. But it is simple model, which actually in practice works out pretty well, OK, you're not going to be able to do more work with P processors than you are with an infinite number of processors.
OK, so those are helpful bounds to understand when we are trying to make something go faster, it's nice to know what you could possibly hope to achieve, OK, as opposed to beating your head against a wall, how come I can't get it to go much faster? Maybe it's because one of these lower bounds is operating. OK, well, we're interested in how fast we can go. That's the main reason for using multiple processors is you hope you're going to go faster than you could with one processor.
So, we define T_1 over T_P to be the speedup on P processors.
OK, so we say, how much faster is it on P processors than on one processor?
OK, that's the speed up. If T_1 over T_P is order P, we say that it's linear speedup.
OK, in other words, why?
Because that says that it means that if I've thrown P processors at the job I'm going to get a speedup that's proportional to P. OK, so when I throw P processors at the job and I get T_P, if that's order P, that means that in some sense my processors each contributed within a constant factor its full measure of support.
If this, in fact, were equal to P, we'd call that perfect linear speedup.
OK, so but here we're looking at giving ourselves, for theoretical purposes, a little bit of a constant buffer here, perhaps. If T_1 over T_P is greater than P, we call that super linear speedup.
OK, so can somebody tell me, when can I get super linear speedup?
When can I get super linear speed up?
Never. OK, why never?
Yeah, if we buy these lower bounds, the first lower bound there, it is T_P is greater than or equal to T_1 over P.
And, if I just take T_1 over T_P, that says it's less than or equal to P. so, this is never, OK, not possible in this model. OK, there are other models where it is possible to get super linear speed up due to caching effects, and things of that nature.
But in this simple model that we are dealing with, it's not possible to get super linear speedup.
OK, not possible. Now, the maximum possible speedup, given some amount of work and critical path length is what? What's the maximum possible speed up I could get over any number of processors?
What's the maximum I could possibly get?
No, I'm saying, no matter how many processors, what's the most speedup that I could get?
T_1 over T infinity, because this is the, so T_1 over T infinity is the maximum I could possibly get.
OK, if I threw an infinite number of processors at the problem, that's going to give me my biggest speedup.
OK, and we call that the parallelism.
OK, so that's defined to be the parallelism.
So the parallelism of the particular algorithm is essentially the work divided by the critical path length.
Another way of viewing it is that this is the average amount of work that can be done in parallel along each step of the critical path. And, we denote it often by P bar. So, do not get confused.
P bar does not have anything to do with P at some level.
OK, P is going to be a certain number of processors you're running. P bar is defined just in terms of the computation you're executing, not in terms of the machine you're running it on. OK, it's just the average amount of work that can be done in parallel along each step of the critical path. OK, questions so far?
So mostly we're just doing definitions so far.
OK, now we get into, OK, so it's helpful to know what the parallelism is, because the parallelism is going to, there's no real point in trying to get speed up bigger than the parallelism. OK, so if you are given a particular computation, you'll be able to say, oh, it doesn't go any faster. You're throwing more processors at it. Why is it that going any faster? And the answer could be, no more parallelism. OK, let's see what I want to, yeah, I think we can raise the example here.
We'll talk more about this model.
Mostly, now, we're going to just talk about DAG's. So, we'll talk about the programming model next time. So, let's talk about scheduling. The goal of scheduler is to map the computation to P processors. And this is typically done by a runtime system, which, if you will, is an algorithm that is running underneath the language layer that I showed you. OK, so the programmer designs an algorithm using spawns, and syncs, and so forth.
Then, underneath that, there's an algorithm that has to actually map that executing program onto the processors of the machine as it executes. And that's the scheduler.
OK, so it's done by the language runtime system, typically. OK, so it turns out that online schedulers, let me just say they're complex.
OK, they're not necessarily easy things to build.
OK, they're not too bad actually.
But, we are not going to go there because we only have two lectures to do this. Instead, we're going to do is we'll illustrate the ideas using off-line scheduling.
OK, so you'll get an idea out of this for what a scheduler does, and it turns out that doing these things online is another level of complexity beyond that.
And typically, the online schedulers that are good, these days, are randomized schedulers.
And they have very strong proofs of their ability to perform. But we're not going to go there. We'll keep it simple.
And in particular, we're going to look at a particular type of scheduler called a greedy scheduler.
So, if you have a DAG to execute, so the basic rules of the scheduler is you can't execute a node until all of the nodes that precede it in the DAG have executed.
OK, so you've got to wait until everything is executed.
So, a greedy scheduler, what it says is let's just try to do as much as possible on every step, OK?
In other words, it says I'm never going to try to guess that it's worthwhile delaying doing something.
If I could do something now, I'm going to do it.
And so, each step is going to correspond to be one of two types. The first type is what we'll call a complete step. And this is a step in which there are at least P threads ready to run.
And, I'm executing on P processors.
There are at least P threads ready to run.
So, what's a greedy strategy here?
I've got P processors. I've got at least P threads.
Run any P. Yeah, first P would be if you had a notion of ordering. That would be perfectly reasonable. Here, we are just going to execute any P. We might make a mistake there, because there may be a particular one that if we execute now, that'll enable more parallelism later on.
We might not execute that one. We don't know.
OK, but basically, what we're going to do is just execute any P willy-nilly. So, there's some, if you will, non-determinism in this step here because which one you execute may or may not be a good choice. OK, the second type of step we're going to have is an incomplete step.
And this is a situation where we have fewer than P threads ready to run. So, what's our strategy there?
Execute all of them. OK, if it's greedy, no point in not executing. OK, so if I've got more than P threads ready to run, I execute any P.
If I have fewer than P threads ready to run, we execute all of them. So, it turns out this is a good strategy. It's not a perfect strategy.
In fact, the strategy of trying to schedule optimally a DAG on P processors is NP complete, meaning it's very difficult.
So, those of you going to take 6.045 or 6.840, I highly recommend these courses, and we'll talk more about that in the last lecture as we talked a little bit about what's coming up in the theory engineering concentration.
You can learn about NP completeness and about how you show that certain problems, there are no good algorithms for them, OK, that we are aware of, OK, and what exactly that means.
So, it turns out that this type of scheduling problem turns out to be a very difficult problem to get it optimal.
But, there's nice theorem, due independently to Graham and Brent. It says, essentially, a greedy scheduler executes any computation, G, with work, T_1, and critical path length, T infinity in time, T_P, less than or equal to T_1 over P plus T infinity -- -- on a computer with P processors.
OK, so, it says that I can achieve T_1 over P plus T infinity. So, what does that say?
If we take a look and compare this with our lower bounds on runtime, how efficient is this? How does this compare with the optimal execution? Yeah, it's two competitive.
It's within a factor of two of optimal because this is a lower bound and this is a lower bound. And so, if I take twice the max of these two, twice the maximum of these two, that's going to be bigger than the sum.
So, I'm within a factor of two of which ever is the stronger, lower bound for any situation. So, this says you get within a factor of two of efficiency of scheduling in terms of the runtime on P processors. OK, does everybody see that?
So, let's prove this theorem. It's quite an elegant theorem.
It's not a hard theorem. One of the nice things, by the way, about this week, is that nothing is very hard.
It just requires you to think differently.
OK, so the proof has to do with counting up how many complete steps we have, and how many incomplete steps we have. OK, so we'll start with the number of complete steps. So, can somebody tell me what's the largest number of complete steps I could possibly have?
Yeah, I heard somebody mumble it back there.
T_1 over P. Why is that?
Yeah, so the number of complete steps is, at most, T_1 over P because why? Yeah, once you've had this many, you've done T_1 work, OK?
So, every complete step I'm getting P work done.
So, if I did more than T_1 over P steps, there would be no more work to be done. So, the number of complete steps can't be bigger than T_1 over P.
OK, so that's this piece. OK, now we're going to count up the incomplete steps, and show its bounded by T infinity. OK, so let's consider an incomplete step. And, let's see what happens.
And, let's let G prime be the subgraph of G that remains to be executed. OK, so we'll draw a picture here. So, imagine we have, let's draw it on a new board.
So here, we're going to have a graph, our graph, G. We're going to do actually P equals three as our example here.
So, imagine that this is the graph, G.
And, I'm not showing the procedures here because this actually is a theorem that works for any DAG.
And, the procedure outlines are not necessary.
All we care about is the threads.
I missed one. OK, so imagine that's my DAG, G, and imagine that I have executed up to this point.
Which ones have I executed? Yeah, I've executed these guys.
So, the things that are in G prime are just the things that have yet to be executed. And these guys are the ones that are already executed. And, we'll imagine that all of them are unit time threads without loss of generality.
The theorem would go through, even if each of these had a particular time associated with it.
The same scheduling algorithm will work just fine.
So, how can I characterize the threads that are ready to be executed? Which are the threads that are ready to be executed here? Let's just see.
So, that one? No, that's not ready to be executed. Why?
Because it's got a predecessor here, this guy.
OK, so this guy is ready to be executed, and this guy is ready to be executed. OK, so those two threads are ready to be, how can I characterize this?
What's their property? What's a graph theoretic property in G prime that tells me whether or not something is ready to be executed? It has no predecessor, but what's another way of saying that?
It's got no predecessor in G prime.
What does it mean for a node not to have a predecessor in a graph? Its in degree is zero, right? Same thing.
OK, the threads with in degree, zero and G prime are the ones that are ready to be executed. OK, and if it's incomplete step, what do I do? I'm going to execute says, if it's an incomplete step, I execute all of them.
OK, so I execute all of these. OK, now I execute all of the in degree zero threads, what happens to the critical path length of the graph that remains to be executed?
It decreases by one. OK, so the critical path length of what remains to be executed, G prime, is reduced by one.
So, what's left to be executed on every incomplete step, what's left to be executed always reduces by one.
Notice the next step here is going to be a complete step, because I've got four things that are ready to go.
And, I can execute them in such a way that the critical path length doesn't get reduced on that step.
OK, but if I had to execute all of them, then it does reduce the critical path length. Now, of course, both could happen, OK, at the same time, OK, but any time that I have an incomplete step, I'm guaranteed to reduce the critical path length by one.
OK, so that implies that the number of incomplete steps is, at most, T infinity. And so, therefore, T of P is, at most, the number of complete steps plus the number of incomplete steps.
And we get our bound. This is sort of an amortized argument if you want to think of it that way, OK, that at every step I'm either amortizing the step against the work, or I'm amortizing it against the critical path length, or possibly both. But I'm at least doing one of those for every step, OK, and so, in the end, I just have to add up the two contributions.
Any questions about that? So this, by the way, is the fundamental theorem of all scheduling.
If ever you study anything having to do with scheduling, this basic result is sort of the foundation of a huge number of things. And then what people do is they gussy it up, like, let's do this online, OK, with a scheduler, etc., that everybody's trying to match these bounds, OK, of what an omniscient greedy scheduler would achieve, OK, and there are all kinds of other things. But this is sort of the basic theorem that just pervades the whole area of scheduling.
OK, let's do a quick corollary. I'm not going to erase those.
Those are just too important. I want to erase those.
Let's not erase those. I want to erase that either.
We're going to go back to the top.
Actually, we'll put the corollary here because that's just one line. OK.
The corollary says you get linear speed up if the number of processors that you allocate, that you run your job on is order, the parallelism. OK, so greedy scheduler gives you linear speed up if you're running on essentially parallelism or fewer processors. OK, so let's see why that is.
And I hope I'll fit this, OK?
So, P bar is T_1 over T infinity.
And that implies that if P equals order T_1 over T infinity, then that says just bringing those around, T infinity is order T_1 over P. So, everybody with me?
It's just algebra. So, it says this is the definition of parallelism, T_1 over T infinity, and so, if P is order parallelism, then it's order T_1 over T infinity. And now, just bring it around.
It says T infinity is order T_1 over P.
So, that says T infinity is order T_1 over P.
OK, and so, therefore, continue the proof here, thus T_P is at most T_1 over P plus T infinity.
Well, if this is order T_1 over P, the whole thing is order T_1 over P. OK, and so, now I have T_P is order T_1 over P, and what we need is to compute T_1 over T_P, and that's going to be order T_P. OK?
Does everybody see that? So what that says is that if I have a certain amount of parallelism, if I run essentially on fewer processors than that parallelism, I get linear speed up if I use greedy scheduling.
OK, if I run on more processors than the parallelism, in some sense I'm being wasteful because I can't possibly get enough speed up to justify those extra processors.
So, understanding parallelism of a job says that's sort of a limit on the number of processors I want to have.
And, in fact, I can achieve that.
Question?
Yeah, really, in some sense, this is saying it should be omega P.
Yeah, so that's fine. It's a question of, so ask again.
No, no, it's only if it's bounded above by a constant.
T_1 and T infinity aren't constants.
They're variables in this. So, we are doing multivariable asymptotic analysis. So, any of these things can be a function of anything else, and can be growing as much as we want. So, the fact that we say we are given it for a particular thing, we're really not given that number. We're given a whole class of DAG's or whatever of various sizes is really what we're talking about. So, I can look at the growth.
Here, where it's talking about the growth of the parallelism, sorry, the growth of the runtime T_P as a function of T_1 and T infinity. So, I am talking about things that are growing here, OK?
OK, so let's put this to work, OK?
And, in fact, so now I'm going to go back to here. Now I'm going to tell you about a little bit of my own research, and how we use this in some of the work that we did. OK, so we've developed a dynamic multithreaded language called Cilk, spelled with a C because it's based on the language, C.
And, it's not an acronym because silk is like nice threads, OK, although at one point my students had a competition for what the acronym silk could mean.
The winner, turns out, was Charles' Idiotic Linguistic Kluge. So anyway, if you want to take a look at it, you can find some stuff on it here. OK, OK, and what it uses is actually one of these more complicated schedulers. It's a randomized online scheduler, OK, and if you look at its expected runtime on P processors, it gets effectively T_1 over P plus O of T infinity provably. OK, and empirically, if you actually look at what kind of runtimes you get to find out what's hidden in the big O there, it turns out, in fact, it's T_1 over P plus T infinity with the constants here being very close to one empirically.
So, no guarantees, but this turns out to be a pretty good bound. Sometimes, you see a coefficient on T infinity that's up maybe close to four or something. But generally, you don't see something that's much bigger than that.
And mostly, it tends to be around, if you do a linear regression curve fit, you get that the constant here is close to one. And so, with this, you get near perfect if you use this formula as a model for your runtime. You get near perfect linear speed up if the number of processors you're running on is much less than your average parallelism, which, of course, is the same thing as if T infinity is much less than T_1 over P. So, what happens here is that when P is much less than P infinity, that is, T infinity is much less than T_1 over P, this term ceases to matter very much, and you get very good speedup, OK, in fact, almost perfect speedup.
So, each processor gives you another processor's work as long as you are the range where the number of processors is much less than the number of parallelism.
Now, with this language many years ago, which seems now like many years ago, OK, it turned out we competed.
We built a bunch of chess programs.
And, among our programs were Starsocrates, and Cilkchess, and we also had several others.
And these were, I would call them, world-class. In particular, we tied for first in the 1995 World Computer Chess Championship in Hong Kong, and then we had a playoff and we lost. It was really a shame.
We almost won, running on a big parallel machine. That was, incidentally, some of you may know about the Deep Blue chess playing program.
That was the last time before they faced then world champion Kasparov that they competed against programs.
They tied for third in that tournament.
OK, so we actually out-placed them.
However, in the head-to-head competition, we lost to them.
So we had one loss in the tournament up to the point of the finals. They had a loss and a draw.
Most people aren't aware that Deep Blue, in fact, was not the reigning World Computer Chess Championship when they faced Kasparov. The reason that they faced Kasparov was because IBM was willing to put up the money.
OK, so we developed these chess programs, and the way we developed them, let me in particular talk about Starsocrates. We had this interesting anomaly come up. We were running on a 32 processor computer at MIT for development.
And, we had access to a 512 processor computer for the tournament at NCSA at the University of Illinois.
So, we had this big machine. Of course, they didn't want to give it to us very much, but we have the same machine, just a small one, at MIT.
So, we would develop on this, and occasionally we'd be able to run on this, and this was what we were developing for on our processor. So, let me show you sort of the anomaly that came up, OK?
So, we had a version of a program that I'll call the original program, OK, and we had an optimized program that included some new features that were supposed to make the program go faster. And so, we timed it on our 32 processor machine. And, it took us 65 seconds to run it. OK, and then we timed this new program. So, I'll call that T prime of sub 32 on our 32 processor machine, and it ran and 40 seconds to do this particular benchmark.
Now, let me just say, I've lied about the actual numbers here to make the calculations easy.
But, the same idea happened. Just the numbers were messier.
OK, so this looks like a significant improvement in runtime, but we rejected the optimization.
OK, and the reason we rejected it is because we understood about the issues of work and critical path.
So, let me show you the analysis that we did, OK? So the analysis, it turns out, if we looked at our instrumentation, the work in this case was 2,048. And, the critical path was one second, which, over here with the optimized program, the work was, in fact, 1,024.
But the critical path was eight.
So, if we plug into our simple model here, the one I have up there with the approximation there, I have T_32 is equal to T_1 over 32 plus T infinity, and that's equal to, well, the work is 2,048 divided by 32.
What's that? 64, good, plus the critical path, one, that's 65. So, that checks out with what we saw. OK, in fact, we did that, and it checked out.
OK, it was very close. OK, over here, T prime of 32 is T prime, one over 32 plus T infinity prime, and that's equal to 1,024 divided by 32 is 32 plus eight, the critical path here. That's 40.
So, that checked out too. So, now what we did is we said is we said, OK, let's extrapolate to our big machine. How fast are these things going to run on our big machine? Well, for that, we want T of 512. And, that's equal to T_1 over 512 plus T infinity. And so, what's 2,048 divided by 512? It's four, plus T infinity is one. That's equal to five.
So, go quite a bit faster on this.
But here, T prime of 512 is equal to T one prime over 512 plus T infinity prime is equal to, well, 1,024 plus divided by 512 is two plus critical path of eight, that's ten.
OK, and so, you see that on the big machine, we would have been running twice as slow had we adopted that, quote, "optimization", OK, because we had run out of parallelism, and this was making the path longer.
We needed to have a way of doing it where we could reduce the work. Yeah, it's good to reduce the work but not as the critical path ends up getting rid of the parallels that we hope to be able to use during the runtime.
So, it's twice as slow, OK, twice as slow.
So the moral is that the work and critical path length predict the performance better than the execution time alone, OK, when you look at scalability.
And a big issue on a lot of these machines is scalability; not always, sometimes you're not worried about scalability.
Sometimes you just care. Had we been running in the competition on a 32 processor machine, we would have accepted this optimization. It would have been a good trade-off. OK, but because we knew that we were running on a machine with a lot more processors, and that we were close to running out of the parallelism, it didn't make sense to be increasing the critical path at that point, because that was just reducing the parallelism of our calculation. OK, next time, any questions about that first? No?
OK. Next time, now that we understand the model for execution, we're going to start looking at the performance of particular algorithms what we code them up in a dynamic, multithreaded style, OK?
Free Downloads
Free Streaming
Video
- iTunes U (MP4 - 157MB)
- Internet Archive (MP4 - 309MB)
Caption
- English-US (SRT)